What Is Accuracy And Precision A Simple Guide
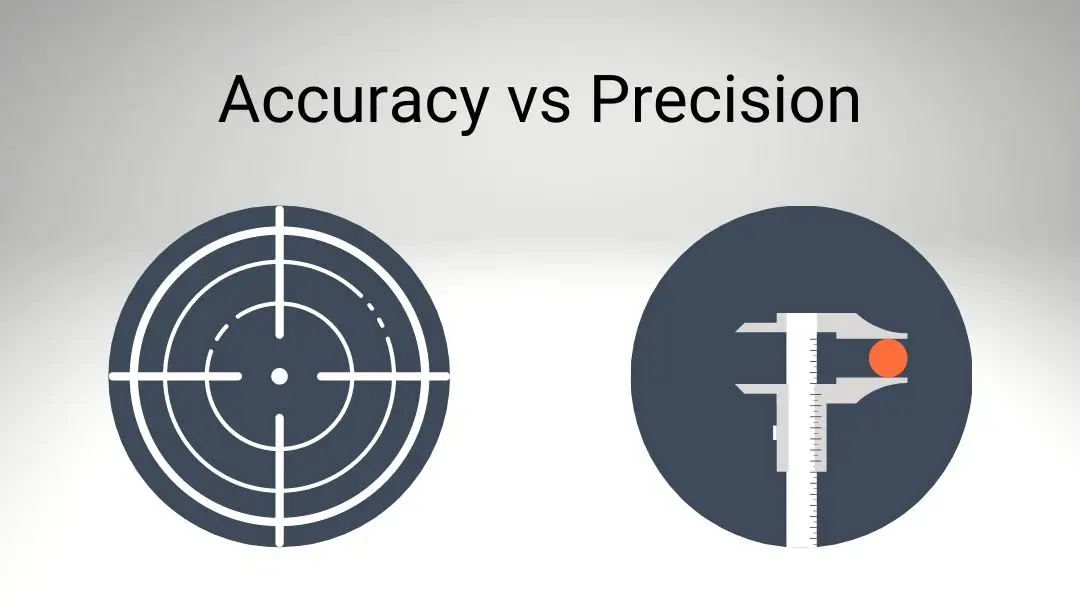
What Is Accuracy And Precision A Simple Guide Both accuracy and precision are the goal of any measurement. variations in accuracy and precision are largely controllable. in target shooting, you improve accuracy by moving closer to the target, or using an aiming aid like a scope or laser pointer. you improve precision by mounting your gun to a table or bench or shooting indoors out of the wind. Tl;dr. accuracy shows how often a classification ml model is correct overall. precision shows how often an ml model is correct when predicting the target class. recall shows whether an ml model can find all objects of the target class. consider the class balance and costs of different errors when choosing the suitable metric.
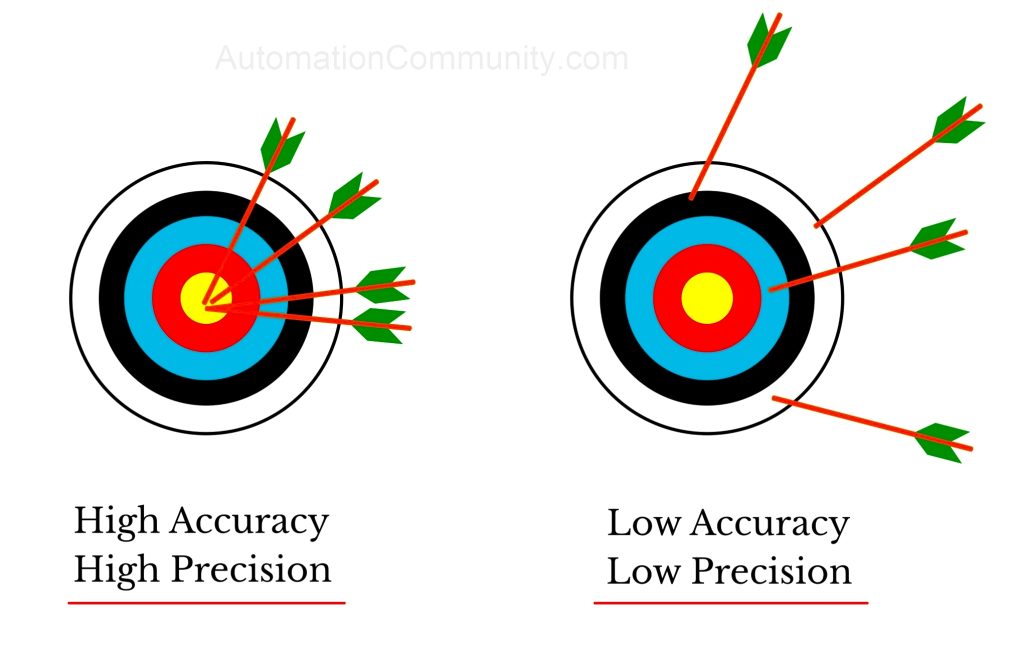
Difference Between Accuracy And Precision Accuracy vs. precision: the key differences. to understand the difference between accuracy and precision, let’s consider a simple analogy involving a target. Illustrating accuracy and precision: the dartboard example. a popular analogy to illustrate the difference between accuracy and precision is the example of a dartboard: high accuracy, low precision: the darts spread out in this scenario. their average position is close to the bullseye (true value). Guide to evaluating logistic regression models: accuracy, precision, recall, and f1 score a confusion matrix is a fundamental tool in evaluating the performance of classification models, including. Both accuracy and f1 (0.51 and 0.02 respectively) are reflecting poor overall performance in this case, but that’s because this is a balanced dataset. in an imbalanced dataset, f1 score but not accuracy will capture a poor balance between recall and precision. that’s f1 score’s use case.
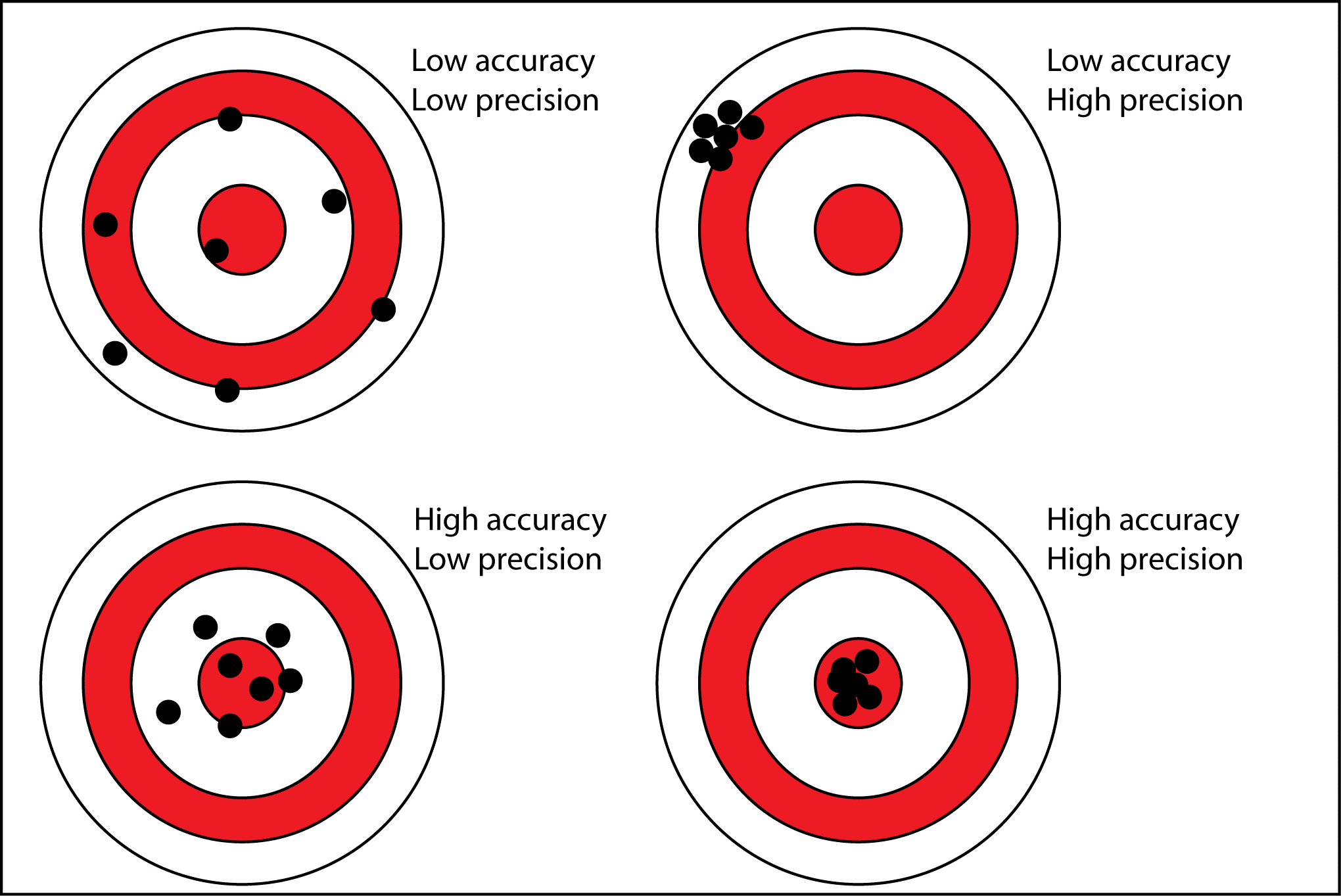
Precision Vs Accuracy Chart Guide to evaluating logistic regression models: accuracy, precision, recall, and f1 score a confusion matrix is a fundamental tool in evaluating the performance of classification models, including. Both accuracy and f1 (0.51 and 0.02 respectively) are reflecting poor overall performance in this case, but that’s because this is a balanced dataset. in an imbalanced dataset, f1 score but not accuracy will capture a poor balance between recall and precision. that’s f1 score’s use case. Both also reflect how close a measurement is to an actual value, but accuracy reflects how close a measurement is to a known or accepted value, while precision reflects how reproducible measurements are, even if they are far from the accepted value. accuracy is how close a value is to its true value. This metric balances the importance of precision and recall, and is preferable to accuracy for class imbalanced datasets. when precision and recall both have perfect scores of 1.0, f1 will also have a perfect score of 1.0. more broadly, when precision and recall are close in value, f1 will be close to their value.
Comments are closed.