Types Of Regression In Data Science Techvidvan

Types Of Regression In Data Science Techvidvan Ln (odds) = ln (p (1 p)) here, p is the probability of the occurrence of the event. () logistic regression requires a large sample size to draw the outcome. 3. polynomial regression. when the relationship between a dependent and independent variable is nonlinear, polynomial regression is used. Non linear regression in r. r non linear regression is a regression analysis method to predict a target variable using a non linear function consisting of parameters and one or more independent variables. non linear regression is often more accurate as it learns the variations and dependencies of the data.
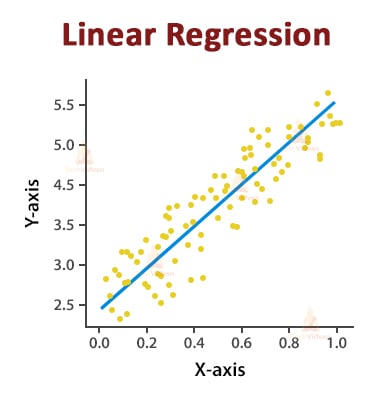
Types Of Regression In Data Science Techvidvan There are three types of logistic regressions in r. these classifications have been made based on the number of values the dependent variable can take. 1. binary logistic regression in r. in binary logistic regression, the target variable or the dependent variable is binary in nature i.e. it has only two possible values. 2. multicollinearity. when the independent variables are highly correlated to each other then the variables are said to be multicollinear. many types of regression techniques assumes multicollinearity should not be present in the dataset. it is because it causes problems in ranking variables based on its importance. 3. polynomial regression. polynomial regression is used to fit a regression model that describes the relationship between one or more predictor variables and a numeric response variable. use when: the relationship between the predictor variable (s) and the response variable is non linear. the response variable is a continuous numeric variable. There you have it! 5 common types of regressions and their properties. all of these regression regularization methods (lasso, ridge and elasticnet) work well in case of high dimensionality and multicollinearity among the variables in the data set. i hope you enjoyed this post and learned something new and useful.
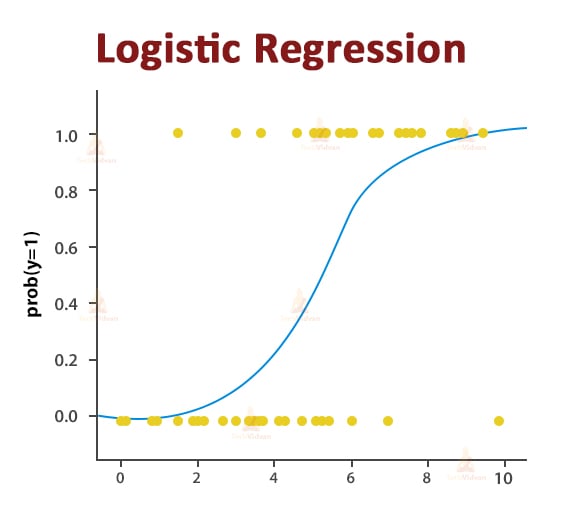
Types Of Regression In Data Science Techvidvan 3. polynomial regression. polynomial regression is used to fit a regression model that describes the relationship between one or more predictor variables and a numeric response variable. use when: the relationship between the predictor variable (s) and the response variable is non linear. the response variable is a continuous numeric variable. There you have it! 5 common types of regressions and their properties. all of these regression regularization methods (lasso, ridge and elasticnet) work well in case of high dimensionality and multicollinearity among the variables in the data set. i hope you enjoyed this post and learned something new and useful. Regression in machine learning consists of mathematical methods that allow data scientists to predict a continuous outcome (y) based on the value of one or more predictor variables (x). linear regression is probably the most popular form of regression analysis because of its ease of use in predicting and forecasting. 6. regression algorithms are widely used in the world of data science and machine learning. in my previous articles, i explained linear and multiple linear regression. but that’s not all. to.
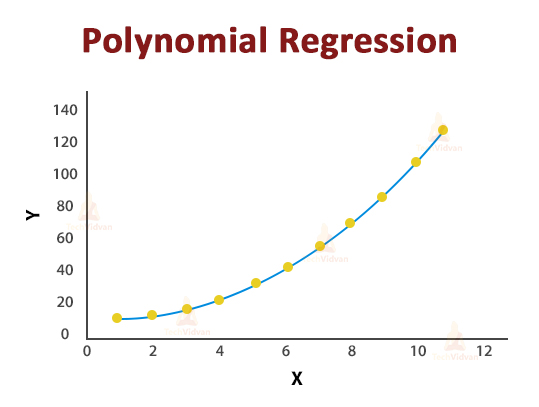
Types Of Regression In Data Science Techvidvan Regression in machine learning consists of mathematical methods that allow data scientists to predict a continuous outcome (y) based on the value of one or more predictor variables (x). linear regression is probably the most popular form of regression analysis because of its ease of use in predicting and forecasting. 6. regression algorithms are widely used in the world of data science and machine learning. in my previous articles, i explained linear and multiple linear regression. but that’s not all. to.
Comments are closed.