The Role Of Mlops In Explainable Ai Use Cases And Approaches
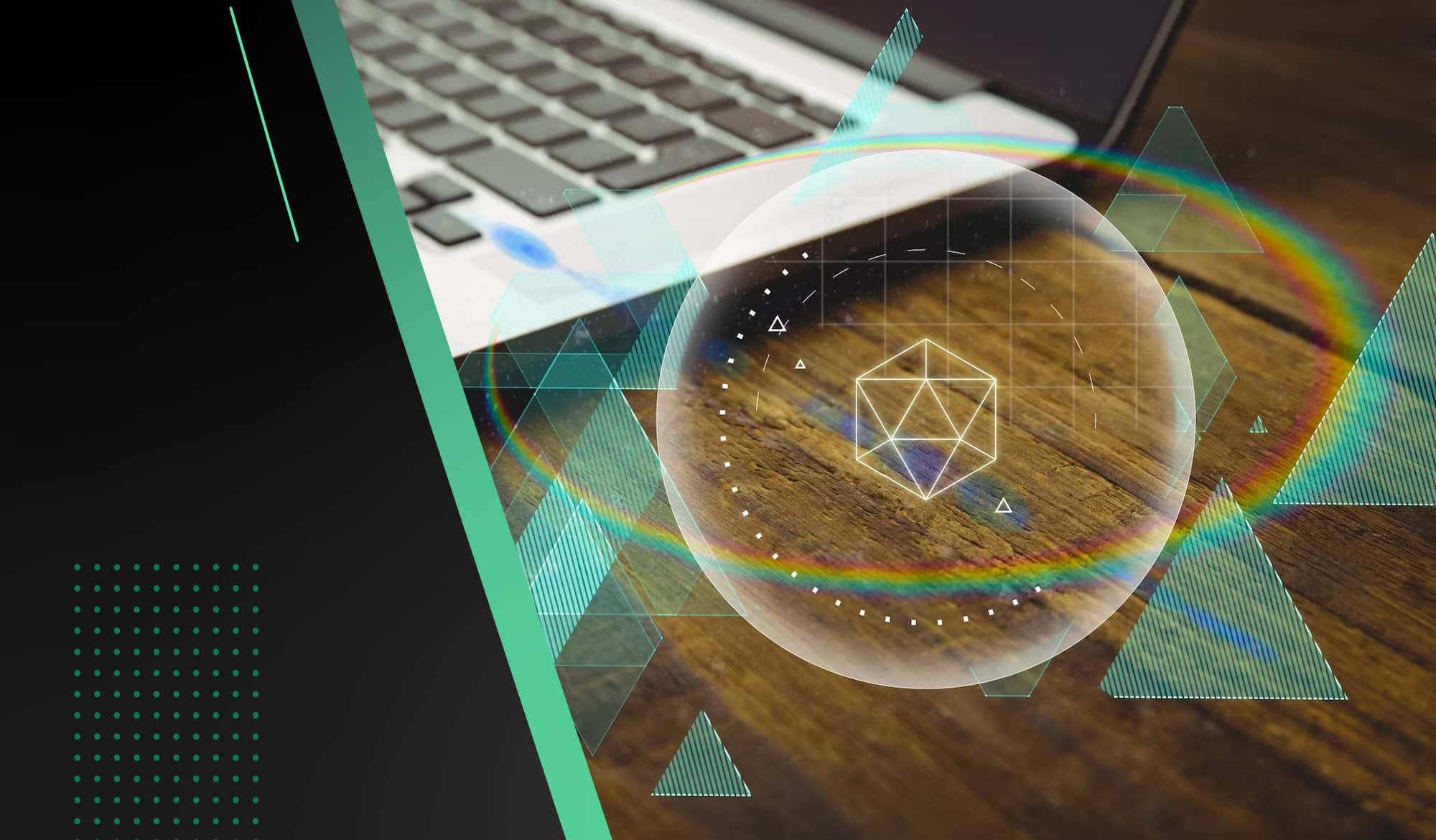
The Role Of Mlops In Explainable Ai Use Cases And Approaches Understanding mlops and its role in xai. mlops (machine learning operations) is a set of practices that enable efficient and scalable development, deployment, and maintenance of machine learning models. mlops can help to create xai models by integrating explainability mechanisms at different stages of the machine learning lifecycle. In recent years, artificial intelligence (ai) technology has been used in most if not all domains and has greatly benefited our lives. while ai can accurately extract critical features and valuable information from large amounts of data to help people complete tasks faster, there are growing concerns about the non transparency of ai in the decision making process. the emergence of explainable.

The Role Of Mlops On Effective Ai By Carl W Handlin Rappi Tech Explainable ai approaches for deep learning encompass a diverse set of techniques designed to elucidate the decision making processes of complex neural network models. fig. 10 illustrates the xai approaches for dl. in this section, these techniques are categorized based on their methodologies and applications in the realm of deep learning. Abstract. this chapter explains the significance of blending two emerging technologies in ai ml—explainable ai (xai) and machine learning operations (ml ops) and demonstrates a focused use case that derives value from leveraging xai to enhance ml ops. the chapter starts by laying out the “growing pains” problems that enterprises are. The ml approaches, which include different mathematical methods for extracting and exploiting important information from huge collections of data, from a technical point of view are now dominated by ai models. the goal of xai research is to make ai systems more comprehensible and transparent to humans without sacrificing performance [20], [21. Explainable ai (xai) explains the inner process of a model i.e., used to provide the explanation of the methods, procedures and output of the processes and that should be understandable by the users. the defense advanced research project agency (darpa) invented the term “explainable ai” (xai). it will be called as “white box” because of.
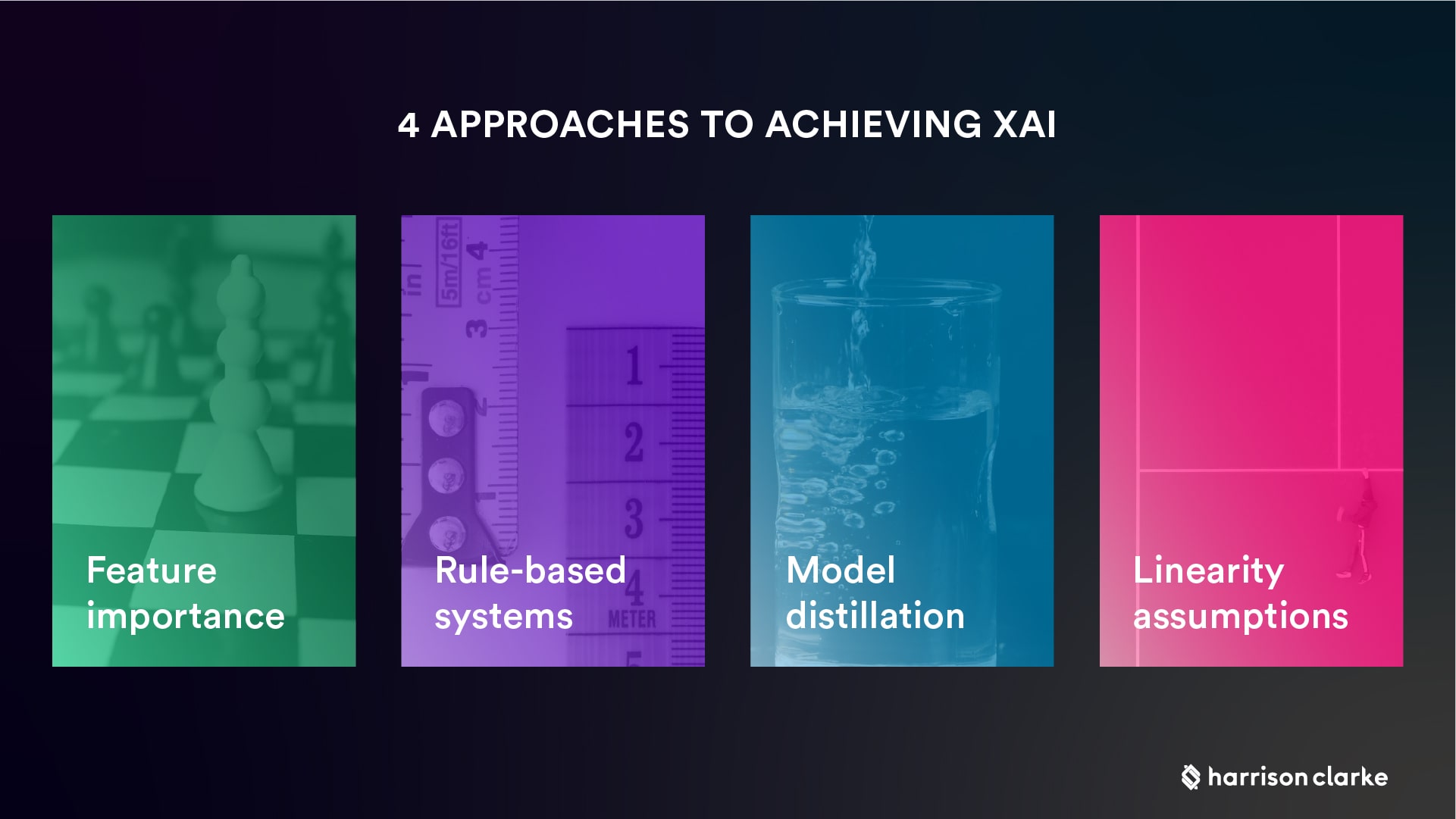
The Role Of Mlops In Explainable Ai Use Cases And Approaches The ml approaches, which include different mathematical methods for extracting and exploiting important information from huge collections of data, from a technical point of view are now dominated by ai models. the goal of xai research is to make ai systems more comprehensible and transparent to humans without sacrificing performance [20], [21. Explainable ai (xai) explains the inner process of a model i.e., used to provide the explanation of the methods, procedures and output of the processes and that should be understandable by the users. the defense advanced research project agency (darpa) invented the term “explainable ai” (xai). it will be called as “white box” because of. Mlops is a set of practices that aims to streamline and automate the lifecycle of ml models in production environments. it's the intersection of ml, devops, and data engineering, designed to make ml systems more reliable, scalable, and maintainable. to understand mlops, it’s crucial to recognize the challenges it addresses. As a result, explainable artificial intelligence (xai) focuses on understanding behind the prediction of ai models to accommodate the demand for transparency in ai tools. interpretability methods of ai models can be classified based on the type of algorithms, the interpretation scale, and the data type [131] .
Comments are closed.