Semantic Segmentation Comparing Models

Semantic Segmentation The Definitive Guide For 2021 Semantic image segmentation (sis) plays a fundamental role in a broad variety of computer vision applications, providing key information for the global understanding of an image. this survey is an effort to summarize two decades of research in the field of sis, where we propose a literature review of solutions starting from early historical methods followed by an overview of more recent deep. **semantic segmentation** is a computer vision task in which the goal is to categorize each pixel in an image into a class or object. the goal is to produce a dense pixel wise segmentation map of an image, where each pixel is assigned to a specific class or object. some example benchmarks for this task are cityscapes, pascal voc and ade20k. models are usually evaluated with the mean.

Semantic Segmentation Models Based On U Net And Deeplabv3 Were Trained The review focuses specifically on semantic segmentation using vision transformers. the comparison of the vit models specialized for semantic segmentation is discussed with architecture wise and tabulated specific sets of model variants that can be compared with the same set of benchmark datasets. The ikshana hypothesis of human scene understanding. 2021. 1. semantic segmentation models are a class of methods that address the task of semantically segmenting an image into different object classes. below you can find a continuously updating list of semantic segmentation models. Several survey papers have been published recently and provide extensive comparison between recent semantic segmentation models for supervised approaches [yyt 18, lr19] as well as semi or weakly supervised methods . one can observe that state of the art methods are currently all based on deep neural networks. We benchmark the robustness of many architectural properties of the state of the art semantic segmenta tion model deeplabv3 for a wide range of real world image corruptions. we utilize almost 400,000 images generated from the cityscapes dataset, pas . cal voc 2012, and ade20k.

Image Segmentation Deep Learning Vs Traditional Guide Several survey papers have been published recently and provide extensive comparison between recent semantic segmentation models for supervised approaches [yyt 18, lr19] as well as semi or weakly supervised methods . one can observe that state of the art methods are currently all based on deep neural networks. We benchmark the robustness of many architectural properties of the state of the art semantic segmenta tion model deeplabv3 for a wide range of real world image corruptions. we utilize almost 400,000 images generated from the cityscapes dataset, pas . cal voc 2012, and ade20k. Segmentation model calibration. eralize to the tasks beyond image classication. in this pa per, we conduct a comprehensive study of the calibration of deep semantic segmentation models. semantic segmentation tags a semantic label to every pixel in an image. over the past years, we have witnessed increasingly accurate dnn models [2,7,39,48,50,52. In open world scenarios, where both novel classes and domains may exist, an ideal segmentation model should detect anomaly classes for safety and generalize to new domains. however, existing methods often struggle to distinguish between domain level and semantic level distribution shifts, leading to poor out of distribution (ood) detection or domain generalization performance. in this work, we.
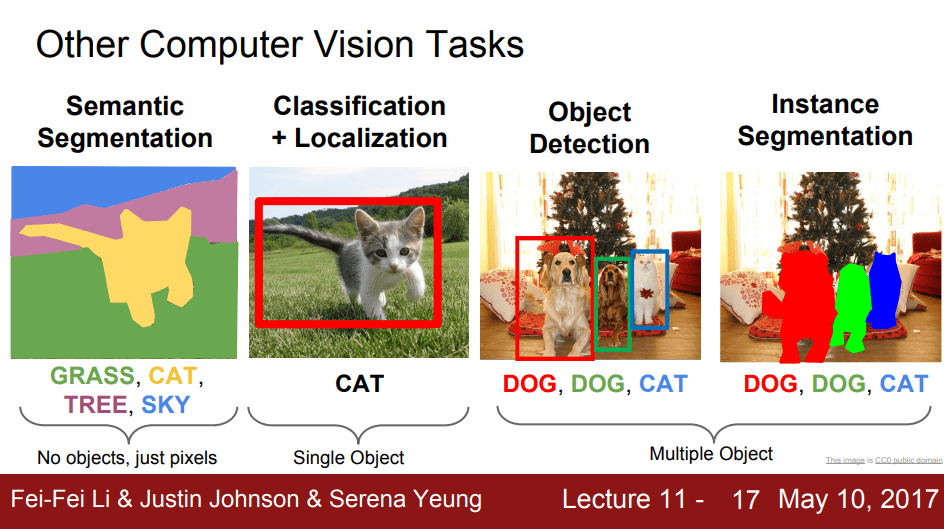
In Depth Guide To Semantic Segmentation Segmentation model calibration. eralize to the tasks beyond image classication. in this pa per, we conduct a comprehensive study of the calibration of deep semantic segmentation models. semantic segmentation tags a semantic label to every pixel in an image. over the past years, we have witnessed increasingly accurate dnn models [2,7,39,48,50,52. In open world scenarios, where both novel classes and domains may exist, an ideal segmentation model should detect anomaly classes for safety and generalize to new domains. however, existing methods often struggle to distinguish between domain level and semantic level distribution shifts, leading to poor out of distribution (ood) detection or domain generalization performance. in this work, we.

Comparative Architecture In Semantic Segmentation A Models Based On
Comments are closed.