Regression 101 Download Free Pdf Coefficient Of Determination
Regression 101 Download Free Pdf Coefficient Of Determination The coefficient of determination is a number between 0 and 1 that measures how well a statistical model predicts an outcome. the model does not predict the outcome. the model partially predicts the outcome. the model perfectly predicts the outcome. the coefficient of determination is often written as r2, which is pronounced as “r squared.”. The regression identity there are three different variations that we can measure. that is, compare y to y. how the y values in the model deviate from their mean. how the y values in the data deviate from the y values in the model. robb t. koether (hampden sydney college) the coefficient of determination wed, apr 17, 2012 7 37.
Coefficient Of Determination Pdf Coefficient Of Determination The coefficient of determination ( Û) is the proportion of the total variation “accounted for” or “explained by” the linear regression of y on x. as proved in appendix 12.2, the coefficient of determination is related to Û and Û by: Û n Û f Û Û l Ú f Û. The coefficient of determination r2 r 2 (or r2 r 2) is the fraction (or percent) of the variation in the values of y y that is explained by the least squares regression of y y on x x. r2 r 2 is a measure of how well the values of y y are explained by x x. for example, there is some variability in the dependent variable values, such as grade. R2 is descriptive statistic which measures the proportion of the “variance” of the dependent variable. y explained by suggested explanatory variables (excluding the constant). however, r2 can be related to a significance test (under the assumptions of the gaussian classical linear model). consider the model. Definition: coefficient of determination. the coefficient of determination of a collection of (x, y) pairs is the number r2 computed by any of the following three expressions: r2 = ssyy − sse ssyy = ss2xy ssxxssyy = β^1 ssxy ssyy. it measures the proportion of the variability in y that is accounted for by the linear relationship between x and y.
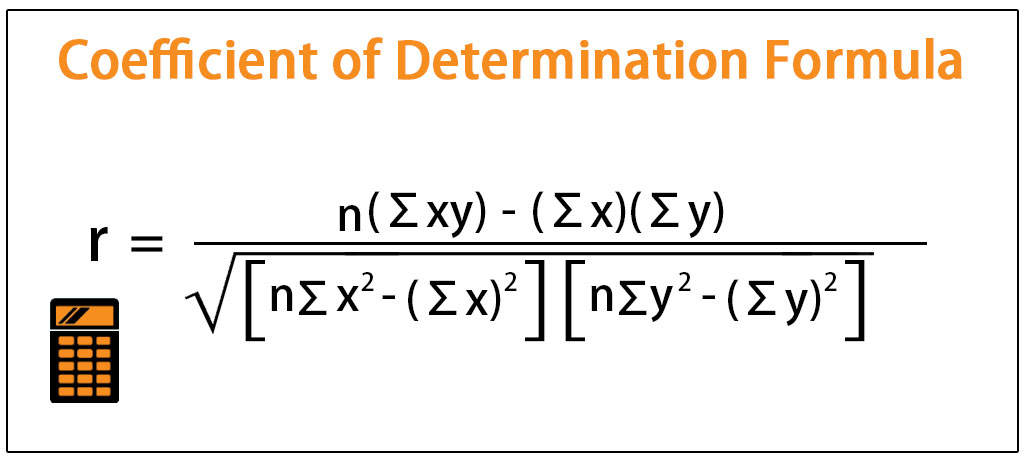
Coefficient Of Determination Definition Example Interpretation R2 is descriptive statistic which measures the proportion of the “variance” of the dependent variable. y explained by suggested explanatory variables (excluding the constant). however, r2 can be related to a significance test (under the assumptions of the gaussian classical linear model). consider the model. Definition: coefficient of determination. the coefficient of determination of a collection of (x, y) pairs is the number r2 computed by any of the following three expressions: r2 = ssyy − sse ssyy = ss2xy ssxxssyy = β^1 ssxy ssyy. it measures the proportion of the variability in y that is accounted for by the linear relationship between x and y. The coefficient of determination allows us to measure the quality of fit of the regression equation to the measured values. to determine the quality of the fit of the regression equation, consider the gap between the observed value and the estimated value for each observation of the sample. this gap (or residual) can also be expressed in the. Upply (the independent variable).as the name implies, linear regression assumes a linear relationship between the depend. nt and the independent variables. the goal is to fit a line to the observations on y and x to minimize the squared deviations from the line; this is the least squares criterion—hence,.
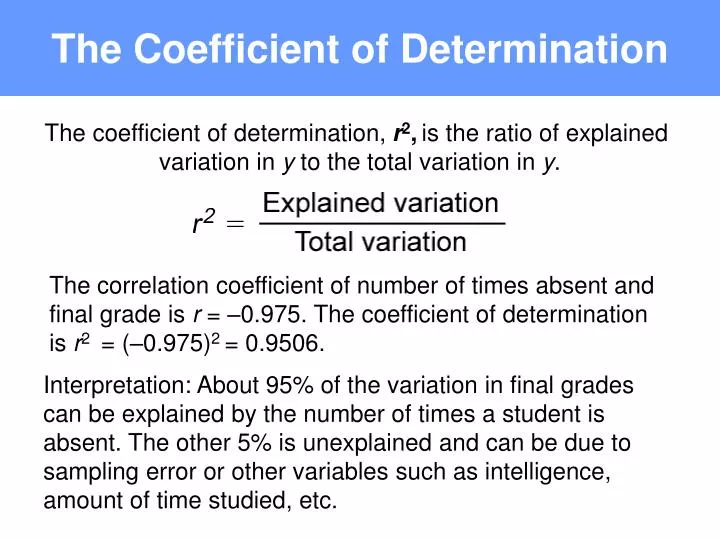
Ppt The Coefficient Of Determination Powerpoint Presentation Free The coefficient of determination allows us to measure the quality of fit of the regression equation to the measured values. to determine the quality of the fit of the regression equation, consider the gap between the observed value and the estimated value for each observation of the sample. this gap (or residual) can also be expressed in the. Upply (the independent variable).as the name implies, linear regression assumes a linear relationship between the depend. nt and the independent variables. the goal is to fit a line to the observations on y and x to minimize the squared deviations from the line; this is the least squares criterion—hence,.
Comments are closed.