Practical Machine Learning Ridge Regression Vs Lasso Hackernoon
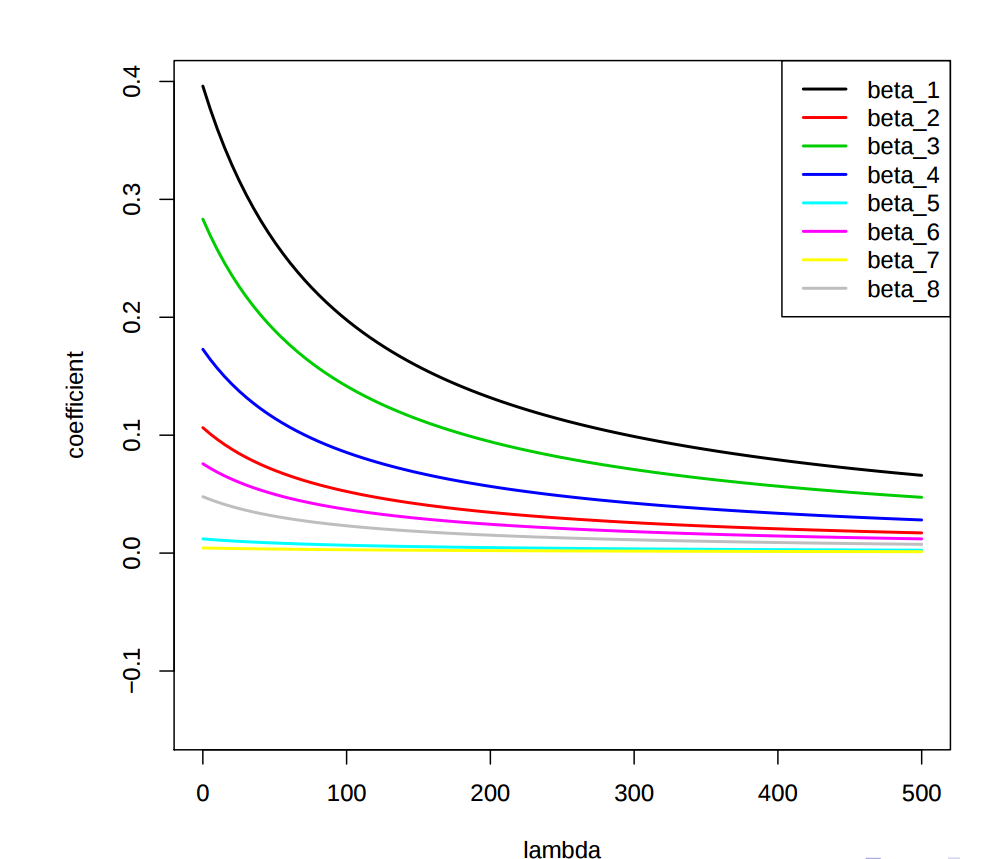
Practical Machine Learning Ridge Regression Vs Lasso Hackernoon Conclusions. machine learning is getting more and more practical and powerful. with zero knowledge in programming, you can train a model to predict house prices in no time. we’ve covered the basics of machine learning, loss function, linear regression, ridge and lasso extensions. This is the most basic form of a loss for a specific data point, that is used mostly for linear regression algorithms. the loss function as a whole can be denoted as: l = ∑ ( Ŷi yi)2. which.

Practical Machine Learning Ridge Regression Vs Lasso By Ofir Chakon Lasso regression. lasso regression uses the l1 penalty term and stands for least absolute shrinkage and selection operator. the penalty applied for l2 is equal to the absolute value of the. Ridge regression adds a penalty equal to the square of the coefficient values. this shrinks the coefficients but doesn’t make any of them exactly zero. while, lasso regression adds a penalty based on the absolute values of the coefficients. this can shrink some coefficients to zero, effectively removing irrelevant features from the model. 1. in the realm of regression analysis, lasso (least absolute shrinkage and selection operator) and ridge regression are two popular techniques used to improve the performance of linear models by. When to use ridge & lasso regression. in ordinary multiple linear regression, we use a set of p predictor variables and a response variable to fit a model of the form: y = β0 β1x1 β2x2 … βpxp ε. the values for β0, β1, b2, … , βp are chosen using the least square method, which minimizes the sum of squared residuals (rss):.

Practical Machine Learning Ridge Regression Vs Lasso Hackernoon 1. in the realm of regression analysis, lasso (least absolute shrinkage and selection operator) and ridge regression are two popular techniques used to improve the performance of linear models by. When to use ridge & lasso regression. in ordinary multiple linear regression, we use a set of p predictor variables and a response variable to fit a model of the form: y = β0 β1x1 β2x2 … βpxp ε. the values for β0, β1, b2, … , βp are chosen using the least square method, which minimizes the sum of squared residuals (rss):. Lasso and ridge (the elements of statistical learning) introduction. when people begin their machine learning journey, they often start with linear regression, one of the most simple algorithms out there. however, this model quickly shows its limitations, especially when working with datasets that lead models to overfit. As was said before, the regression model that use l1 regularization technique is lasso regression and model which uses l2 regularization technique is ridge regression. the key difference between these two is the penalty term. ridge regression adds “square magnitude” of coefficient as penalty term to the loss function. the second part of the.

Ridge Vs Lasso Regression Visualized Youtube Lasso and ridge (the elements of statistical learning) introduction. when people begin their machine learning journey, they often start with linear regression, one of the most simple algorithms out there. however, this model quickly shows its limitations, especially when working with datasets that lead models to overfit. As was said before, the regression model that use l1 regularization technique is lasso regression and model which uses l2 regularization technique is ridge regression. the key difference between these two is the penalty term. ridge regression adds “square magnitude” of coefficient as penalty term to the loss function. the second part of the.
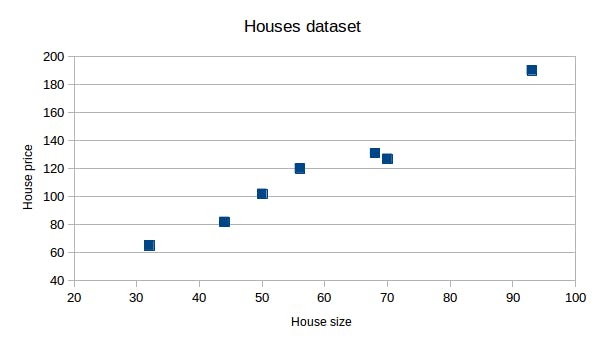
Practical Machine Learning Ridge Regression Vs Lasso Hackernoon
Comments are closed.