Pdf Federated Deep Reinforcement Learning Based Caching And Bitrate

Pdf Federated Deep Reinforcement Learning Based Caching And Bitrate Based on the proposed hc mec network model, this paper constructs a federated deep reinforcement learning based caching and bitrate adaptation scheme, which is shown in figure 3. in this framework, the fdrl cba algorithm proposed in this paper is implemented based on the clustered edge. The federated deep reinforcement learning (fdrl) algorithm is proposed to solve the problem of caching and bitrate adaptation (called fdrl cba) for vr panoramic video services. the simulation results show that fdrl cba can outperform existing drl based methods in the same scenarios in terms of cache hit rate and quality of experience (qoe).

Pdf Feddrl Deep Reinforcement Learning Based Adaptive Aggregation Algorithms, such as deep q learning, advantage actor critic, and proxi mal policy optimization, yield better performance than the traditional bitrate adaptation methods in various environments. keywords: bitrate adaptation · deep reinforcement learning · federated learning · dynamic adaptive streaming over http 1 introduction. The simulations show that the federated drl based rate adaptations, called fdrlabr with different drl algorithms, such as deep q learning, advantage actor critic, and proximal policy optimization, yield better performance than the traditional bitrate adaptation methods in various environments. in video streaming over http, the bitrate adaptation selects the quality of video chunks depending on. Intelligence (ai) based methods, such as deep reinforcement learning (drl), can utilize data driven intelligent decision making and optimization techniques to automatically learn and adjust caching strategies to adapt to evolving data access pat terns and network conditions. this makes them more feasible and effective for practical applications. In this paper, we propose a cooperative edge caching scheme based on elastic federated learning and multi agent deep reinforcement learning (cefmr) scheme to optimize the cost of fetching contents in the next generation network. the contributions of this paper are summarized as follows1.

Pdf Service Based Federated Deep Reinforcement Learning For Anomaly Intelligence (ai) based methods, such as deep reinforcement learning (drl), can utilize data driven intelligent decision making and optimization techniques to automatically learn and adjust caching strategies to adapt to evolving data access pat terns and network conditions. this makes them more feasible and effective for practical applications. In this paper, we propose a cooperative edge caching scheme based on elastic federated learning and multi agent deep reinforcement learning (cefmr) scheme to optimize the cost of fetching contents in the next generation network. the contributions of this paper are summarized as follows1. Abstract—in this paper, the placement strategy design of coded caching in fog radio access networks (f rans) is investigated. by considering time variant content popularity, federated deep re inforcement learning is exploited to learn the placement strategy for our coded caching scheme. In video streaming over http, the bitrate adaptation selects the quality of video chunks depending on the current network condition. some previous works have applied deep reinforcement learning (drl) algorithms to determine the chunk's bitrate from the observed states to maximize the quality of experience (qoe). however, to build an intelligent model that can predict in various environments.

Pdf Federated Deep Reinforcement Learning Based Bitrate Adaptation Abstract—in this paper, the placement strategy design of coded caching in fog radio access networks (f rans) is investigated. by considering time variant content popularity, federated deep re inforcement learning is exploited to learn the placement strategy for our coded caching scheme. In video streaming over http, the bitrate adaptation selects the quality of video chunks depending on the current network condition. some previous works have applied deep reinforcement learning (drl) algorithms to determine the chunk's bitrate from the observed states to maximize the quality of experience (qoe). however, to build an intelligent model that can predict in various environments.
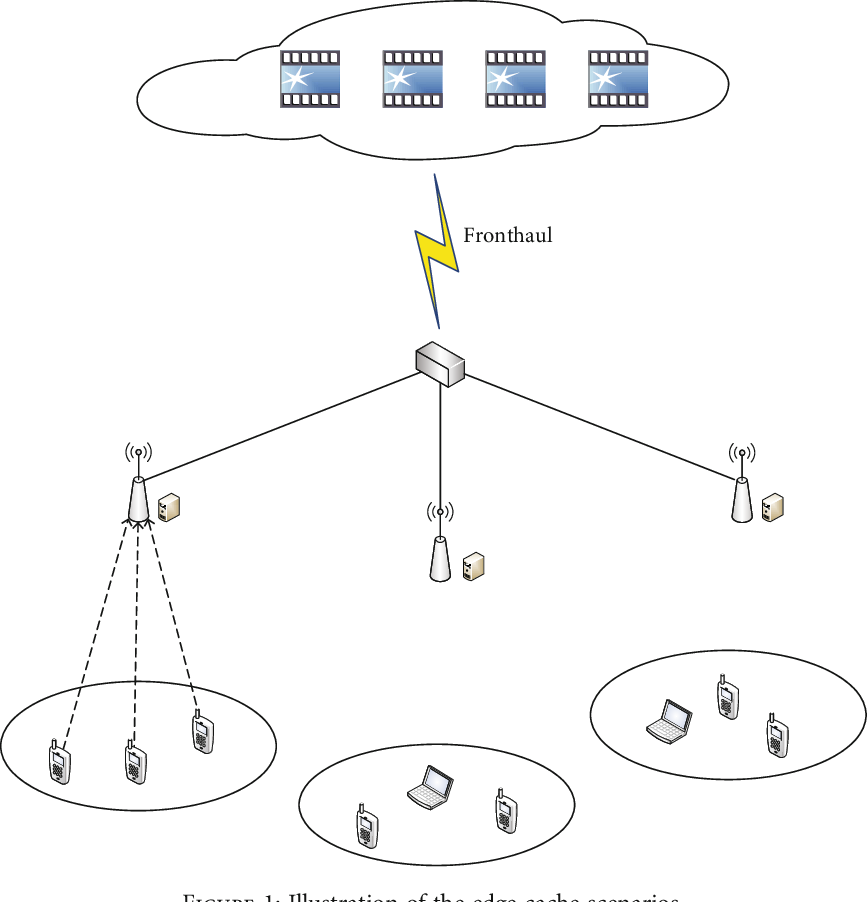
Pdf Collaborative Caching In Edge Computing Via Federated Learning

Pdf Joint Microservices Caching And Task Offloading Framework In Vec
Comments are closed.