Mlops For Machine Learning Implementation Aspire Systems

Mlops For Machine Learning Implementation Aspire Systems Mlops (machine learning operations) is a framework bringing together guiding principles and best practices from machine learning, data engineering, devops to streamline and bring together the reliable and efficient deployment and production of ml models. an analogy to bring the picture of mlops is to compare what it has done for ml as the. Mlops stands for machine learning operations. it is a set of practices that combines devops, data engineering, and machine learning. mlops aims to deploy and maintain ml models reliably and efficiently. properly defined, mlops is an engineering discipline, which seeks to unify ml systems development and deployment to streamline the delivery of.

Mlops For Machine Learning Implementation Aspire Systems This document discusses techniques for implementing and automating continuous integration (ci), continuous delivery (cd), and continuous training (ct) for machine learning (ml) systems. data science and ml are becoming core capabilities for solving complex real world problems, transforming industries, and delivering value in all domains. Mlops is crucial for optimizing and automating machine learning processes in production. it involves deploying, monitoring, and governing ml operations to maximize benefits and mitigate risks. automated model retraining and timely detection of model drift are becoming standard practices. Getting started with mlops and machine learning. if you’re looking to adopt mlops and want to learn more before you start building, you should start with the following: the four ways of doing machine learning: understand the different ways to adopt machine learning at a high level. mlops for research teams: understand why mlops isn’t just. Mlops aims to bridge the gap between the experimental nature of machine learning and the operational requirements of production systems, ensuring that ml models are not only developed efficiently.

Unlocking The Power Of Mlops Managing The Lifecycle Of Machine By Getting started with mlops and machine learning. if you’re looking to adopt mlops and want to learn more before you start building, you should start with the following: the four ways of doing machine learning: understand the different ways to adopt machine learning at a high level. mlops for research teams: understand why mlops isn’t just. Mlops aims to bridge the gap between the experimental nature of machine learning and the operational requirements of production systems, ensuring that ml models are not only developed efficiently. This is the perfect mlops roadmap guide for you. in recent years, machine learning operations (mlops) has emerged as one of the most sought after fields in the tech industry. with businesses increasingly relying on data driven solutions, mlops professionals are in high demand to deploy and manage machine learning models effectively. Mlops principles. as machine learning and ai propagate in software products and services, we need to establish best practices and tools to test, deploy, manage, and monitor ml models in real world production. in short, with mlops we strive to avoid “technical debt” in machine learning applications. sig mlops defines “an optimal mlops.
How To Implement Mlops This is the perfect mlops roadmap guide for you. in recent years, machine learning operations (mlops) has emerged as one of the most sought after fields in the tech industry. with businesses increasingly relying on data driven solutions, mlops professionals are in high demand to deploy and manage machine learning models effectively. Mlops principles. as machine learning and ai propagate in software products and services, we need to establish best practices and tools to test, deploy, manage, and monitor ml models in real world production. in short, with mlops we strive to avoid “technical debt” in machine learning applications. sig mlops defines “an optimal mlops.
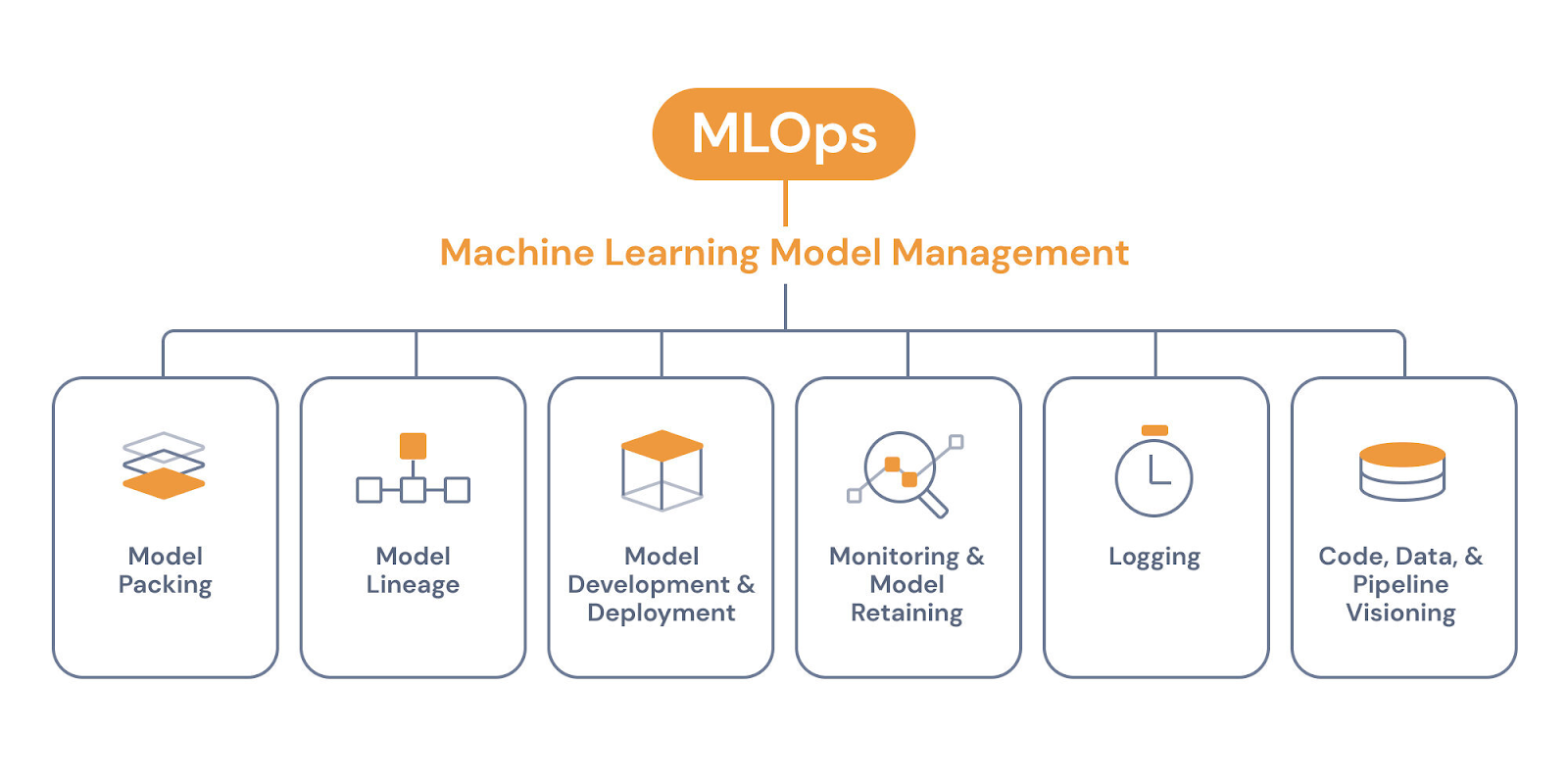
What Is Mlops And Their Various Training Programs Articles Blog
Comments are closed.