Mlops Explainability Ensuring Transparency Accountability In Ai
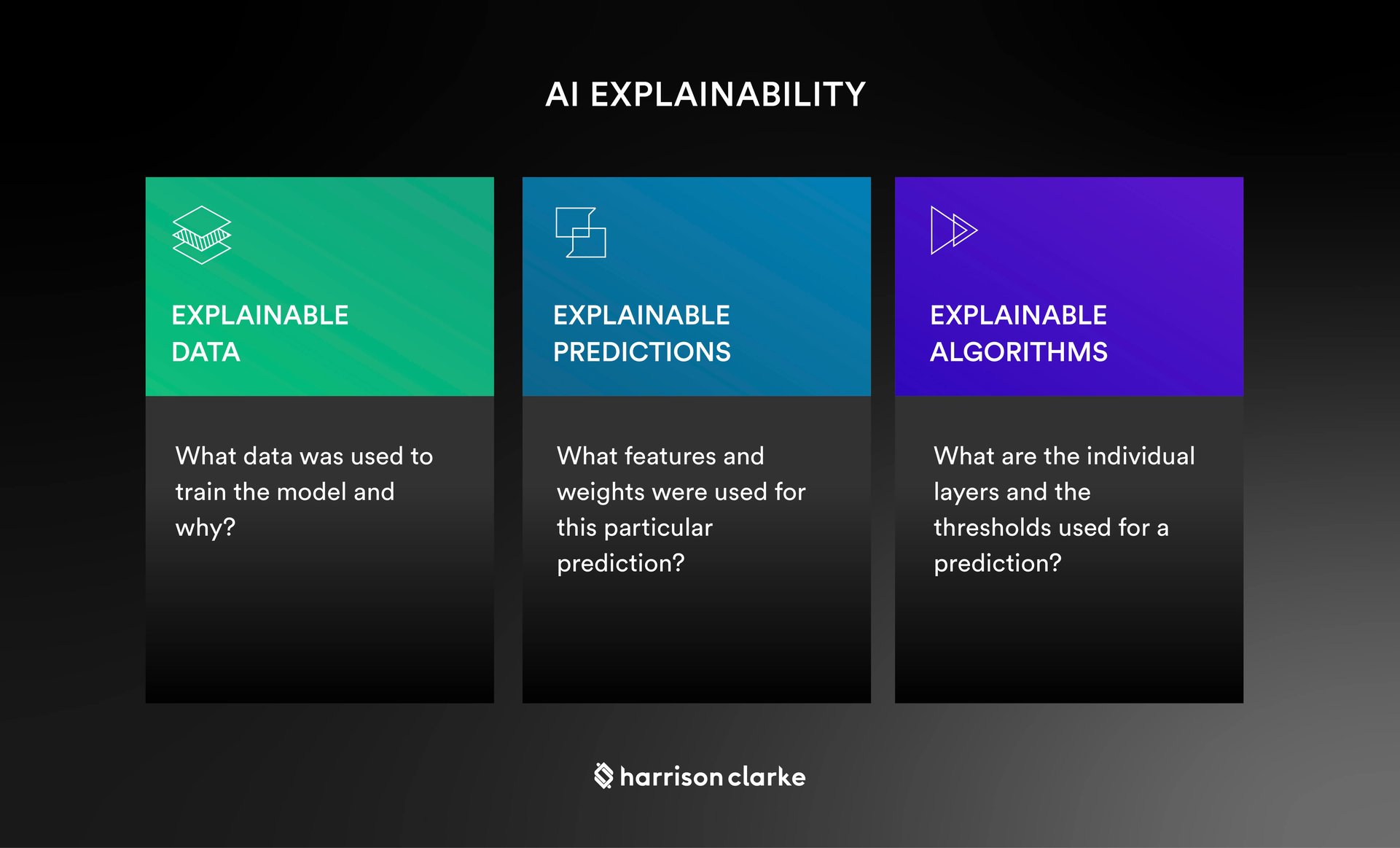
Mlops Explainability Ensuring Transparency Accountability In Ai Ai is used to automate a variety of tasks, from data analysis to customer service. but with the rise of ai comes the need for transparency and accountability. this is where mlops and explainability come into play. mlops, or machine learning operations, is a process for managing the development, deployment, and maintenance of machine learning. Understanding mlops and its role in xai. mlops (machine learning operations) is a set of practices that enable efficient and scalable development, deployment, and maintenance of machine learning models. mlops can help to create xai models by integrating explainability mechanisms at different stages of the machine learning lifecycle.
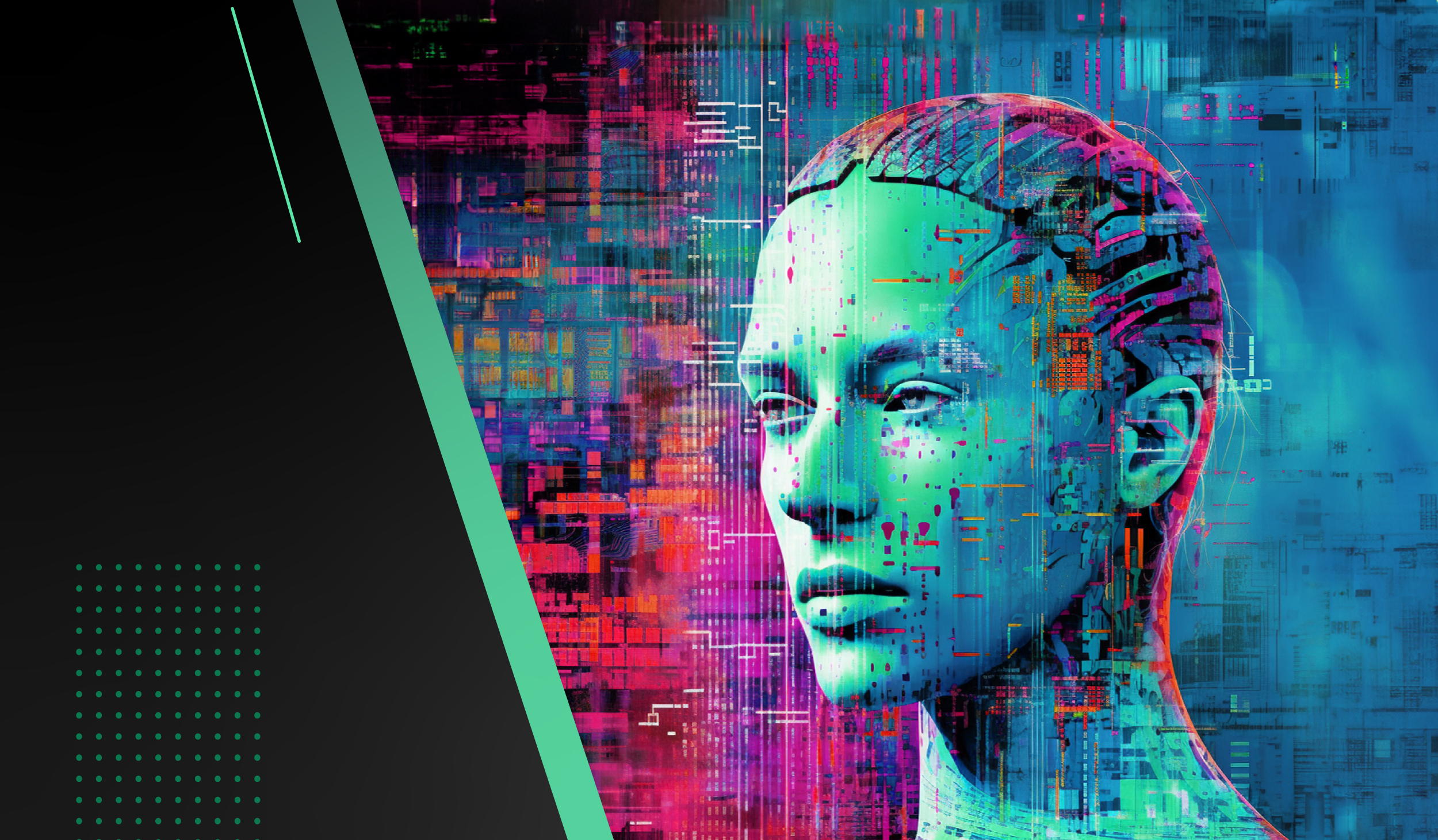
Mlops Explainability Ensuring Transparency Accountability In Ai The intricate relation between explainability, interpretability, and transparency forms a harmonious equation in the realm of responsible ai. transparency serves as the main idea, involving the. Thus, understandability supports explainability and transparency by ensuring the utilization of ai is conveyed to people clearly and in necessary detail. traceability in transparency guidelines accentuates the importance of tracing the decisions of the ai systems (o2, o12). Advances in model explainability and transparency. the demand for explainable ai is driving advances in model interpretability tools and techniques. future mlops practices will likely include more sophisticated methods for ensuring transparency and accountability in machine learning models. growth of automated machine learning (automl). Reliability and robustness: mlops automates the detection of data drift and triggers model retraining, ensuring that ai systems remain reliable over time, even as real world data changes. this combination of automated retraining and human expertise ensures that models continue to meet performance expectations in dynamic environments.
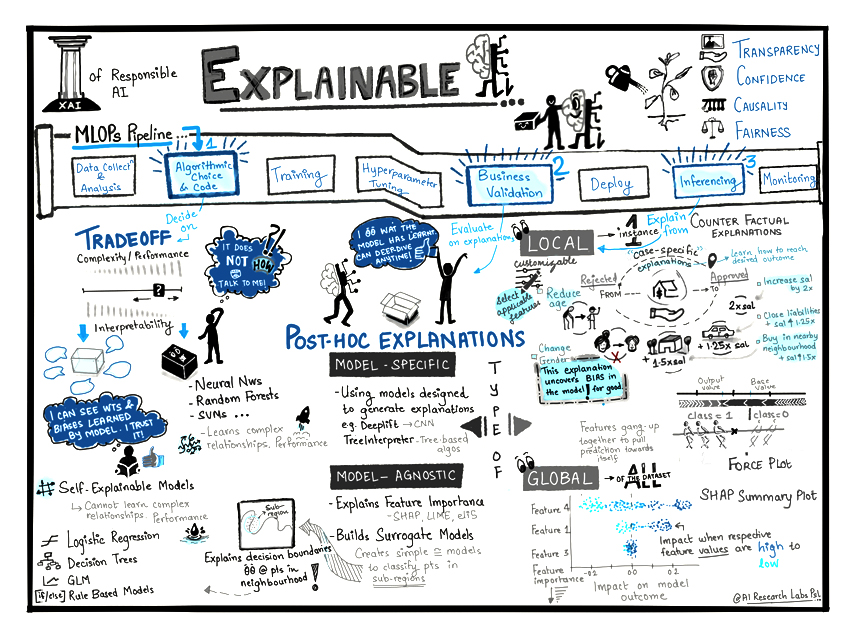
Simplifying Role Of Explainability In The Mlops Cycle Advances in model explainability and transparency. the demand for explainable ai is driving advances in model interpretability tools and techniques. future mlops practices will likely include more sophisticated methods for ensuring transparency and accountability in machine learning models. growth of automated machine learning (automl). Reliability and robustness: mlops automates the detection of data drift and triggers model retraining, ensuring that ai systems remain reliable over time, even as real world data changes. this combination of automated retraining and human expertise ensures that models continue to meet performance expectations in dynamic environments. Mlops is driven by automation and metrics. quality and reliability, for instance, are measured and monitored through metrics like mean time to restore and change failure rate (percentage of deployments causing failure in production). in machine learning, metrics guide training and testing. in trustworthy ai, metrics serve a dual purpose. The need for explainability in ai models is a key aspect of trusted ai, and the lack of explainability can have significant regulatory and ethical implications. as ai continues to play an increasingly important role in various domains of human life, ensuring that ai systems are trustworthy and transparent will become increasingly important.
Comments are closed.