Mle Vs Ols Maximum Likelihood Vs Least Squares In Linear Regression

Mle Vs Ols Maximum Likelihood Vs Least Squares In Linear Regression 16. ml is a higher set of estimators which includes least absolute deviations (l1 l 1 norm) and least squares (l2 l 2 norm). under the hood of ml the estimators share a wide range of common properties like the (sadly) non existent break point. in fact you can use the ml approach as a substitute to optimize a lot of things including ols as. See all my videos at: tilestats at 9:03 i should have said 4.24 and not 4.25.1. ordinary least squares (0:30) 2. maximum likelihood estimatio.

Mle Vs Ols Estimate Mp4 Youtube That is, the difference is in the denominator. however, to construct the v: ˆσ2 ols ∗ (n − 2) σ2 = ∑ni = 1 (yi – ˆβ0 − ˆβ1xi)2 σ2 = ˆσ2 mle ∗ n σ2. thus, ols and mle actually get the same v. beyong that, ols and mle get the same z0 and z1. thus, ols and mle will generate the same t statistic. 16. when is it preferable to use maximum likelihood estimation instead of ordinary least squares? what are the strengths and limitations of each? i am trying to gather practical knowledge on where to use each in common situations. regression. maximum likelihood. least squares. share. cite. 2.1 ols. in this first chapter we will dive a bit deeper into the methods outlined in the video "what is maximum likelihood estimation (w regression). in the video, we touched on one method of linear regression, least squares regression. recall the general intuition is that we want to minimize the distance each point is from the line. Maximum likelihood estimator(s) 1. 0 b 0 same as in least squares case 2. 1 b 1 same as in least squares case 3. ˙ 2 ˙^2 = p i (y i y^ i)2 n 4.note that ml estimator is biased as s2 is unbiased and s2 = mse = n n 2 ^˙2.
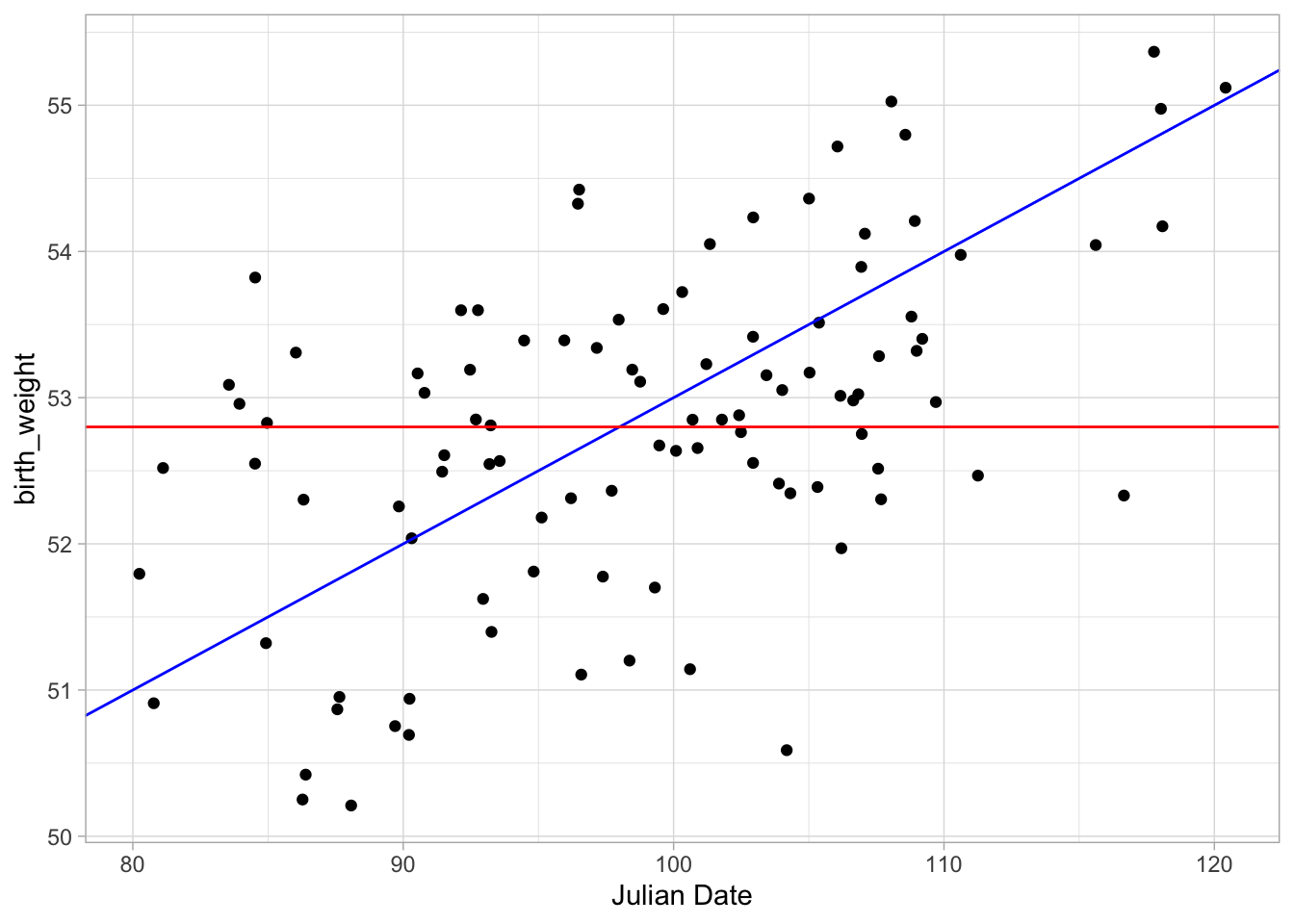
Chapter 2 Linear Regression By Ols And Mle Abracadabra Stats 2.1 ols. in this first chapter we will dive a bit deeper into the methods outlined in the video "what is maximum likelihood estimation (w regression). in the video, we touched on one method of linear regression, least squares regression. recall the general intuition is that we want to minimize the distance each point is from the line. Maximum likelihood estimator(s) 1. 0 b 0 same as in least squares case 2. 1 b 1 same as in least squares case 3. ˙ 2 ˙^2 = p i (y i y^ i)2 n 4.note that ml estimator is biased as s2 is unbiased and s2 = mse = n n 2 ^˙2. Maximum likelihood estimation. the likelihood function can be maximized w.r.t. the parameter(s) Θ, doing this one can arrive at estimators for parameters as well. l({xi }n i=1 , Θ) =. i=1 n f (xi ; Θ) • to do this, find solutions to (analytically or by following gradient) dl({x i }n ,Θ) i=1 = 0. dΘ. The results of this process however, are well known to reach the same conclusion as ordinary least squares (ols) regression [2]. this is because ols simply minimises the difference between the predicted value and the actual value: which is the same result as for maximum likelihood estimation!.
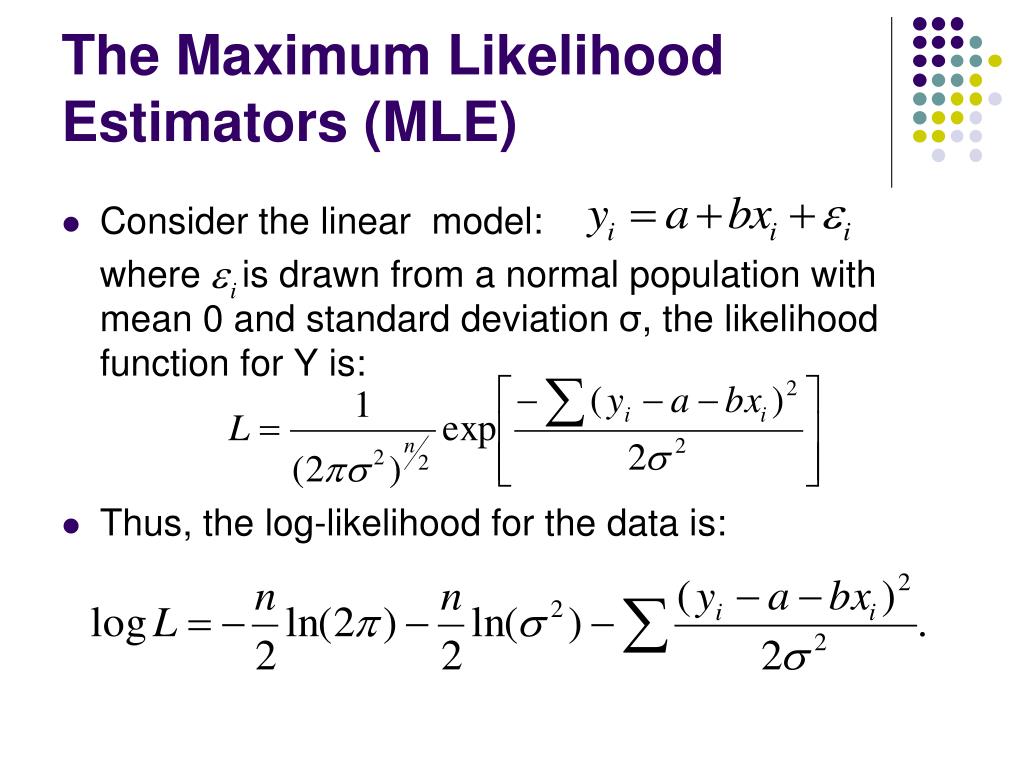
Ppt Simple Linear Regression Powerpoint Presentation Free Download Maximum likelihood estimation. the likelihood function can be maximized w.r.t. the parameter(s) Θ, doing this one can arrive at estimators for parameters as well. l({xi }n i=1 , Θ) =. i=1 n f (xi ; Θ) • to do this, find solutions to (analytically or by following gradient) dl({x i }n ,Θ) i=1 = 0. dΘ. The results of this process however, are well known to reach the same conclusion as ordinary least squares (ols) regression [2]. this is because ols simply minimises the difference between the predicted value and the actual value: which is the same result as for maximum likelihood estimation!.
Comments are closed.