Main Steps Of The Construction Of The Six Machine Learning Models To

Main Steps Of The Construction Of The Six Machine Learning Models To Step 1: data collection for machine learning. data collection is a crucial step in the creation of a machine learning model, as it lays the foundation for building accurate models. in this phase of machine learning model development, relevant data is gathered from various sources to train the machine learning model and enable it to make. You’ve taken a major step into the world of data science by understanding the core steps involved in building a machine learning model from scratch. remember, this is just the beginning.
Main Steps Of The Construction Of The Six Machine Learning Models To The six steps to building a machine learning model include: contextualise machine learning in your organisation. explore the data and choose the type of algorithm. prepare and clean the dataset. split the prepared dataset and perform cross validation. perform machine learning optimisation. deploy the model. Whether you’re a beginner or looking to refine your skills, this guide will walk you through the essential steps of building a machine learning model, from data preprocessing to model evaluation. step 1: define the problem. the first and most crucial step in building a machine learning model is to clearly define the problem you’re trying to. For example, if n is equal to 30 then there are 30 folds (1 sample per fold). as in any other n fold cv, 1 fold is left out as the testing set while the remaining 29 folds are used to build the model. next, the built model is applied to make prediction on the left out fold. Step 7. iterate and adjust the model in production. it's often said that the formula for success when implementing technologies is to start small, think big and iterate often. even after a machine learning model is in production and you're continuously monitoring its performance, you're not done.
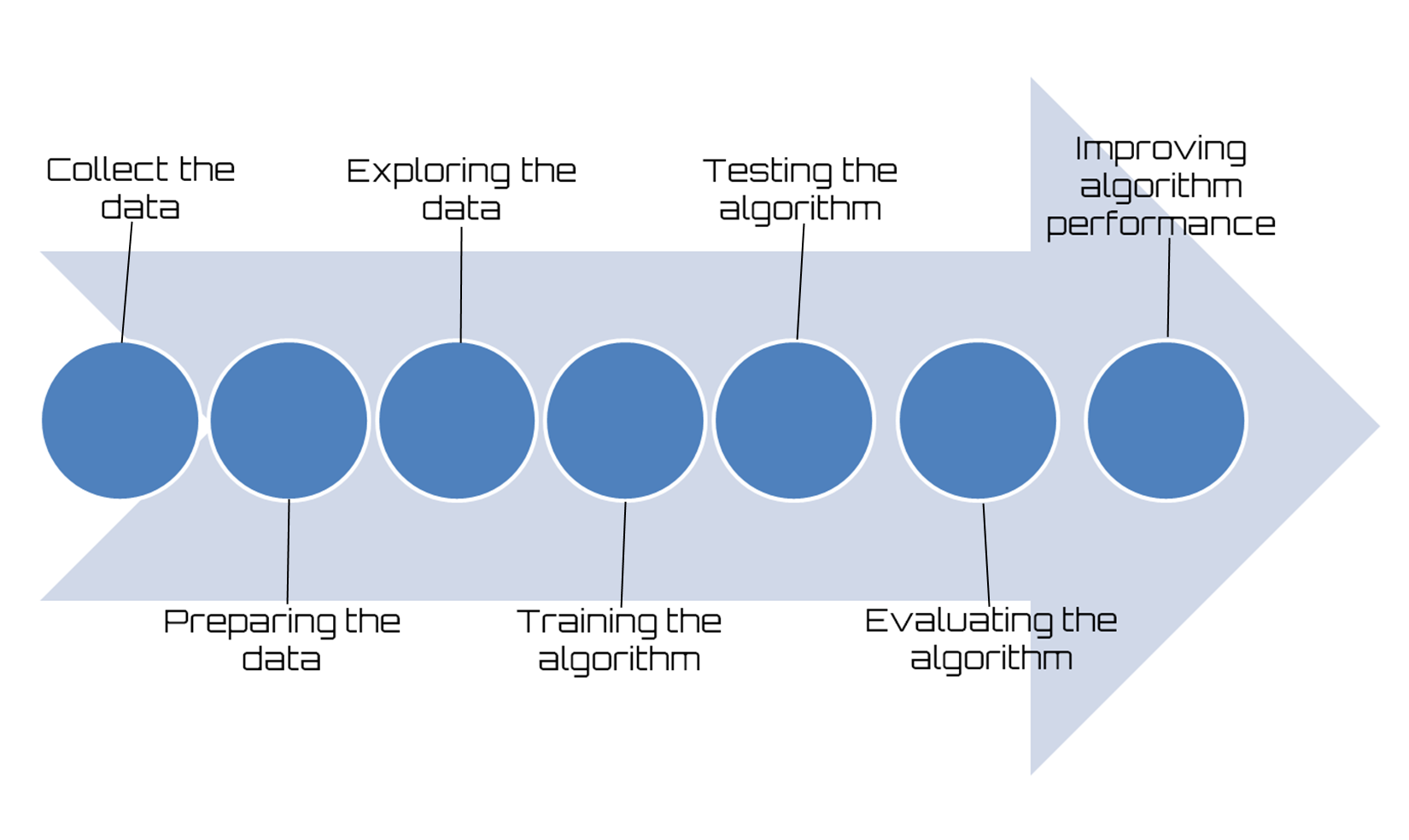
Machine Learning Model Building Steps For example, if n is equal to 30 then there are 30 folds (1 sample per fold). as in any other n fold cv, 1 fold is left out as the testing set while the remaining 29 folds are used to build the model. next, the built model is applied to make prediction on the left out fold. Step 7. iterate and adjust the model in production. it's often said that the formula for success when implementing technologies is to start small, think big and iterate often. even after a machine learning model is in production and you're continuously monitoring its performance, you're not done. We usually think that machine learning (ml) projects involve data processing, model training, and model deployment. but it is so much more than that. we need business and data understanding, data collection techniques, data analytics, model building, and model evaluation. furthermore, after deployment, we need constant monitoring and maintenance. Image by author. 1. accuracy: accuracy can be defined as the fraction of correct predictions made by the machine learning model. the formula to calculate accuracy is: in this case, the accuracy is 46, or 0.67. 2. precision: precision is a metric used to calculate the quality of positive predictions made by the model.

Machine Learning Model Building Steps We usually think that machine learning (ml) projects involve data processing, model training, and model deployment. but it is so much more than that. we need business and data understanding, data collection techniques, data analytics, model building, and model evaluation. furthermore, after deployment, we need constant monitoring and maintenance. Image by author. 1. accuracy: accuracy can be defined as the fraction of correct predictions made by the machine learning model. the formula to calculate accuracy is: in this case, the accuracy is 46, or 0.67. 2. precision: precision is a metric used to calculate the quality of positive predictions made by the model.
Comments are closed.