Machine Learning Model Building Steps
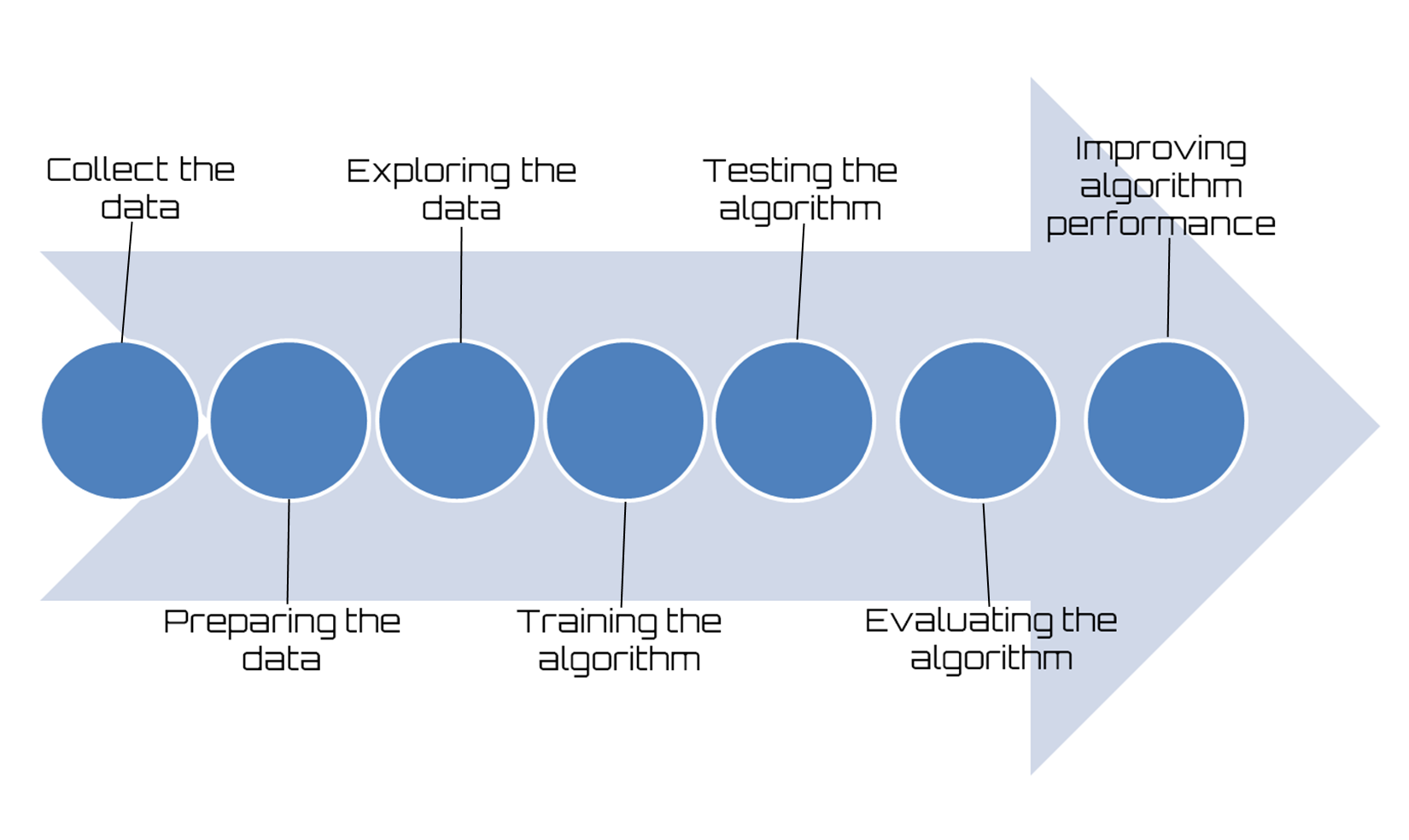
How To Build Machine Learning Models Step By Step Matlab For Machine Table of content. understanding the fundamentals of machine learning. comprehensive guide to building a machine learning model. step 1: data collection for machine learning. step 2: data preprocessing and cleaning. step 3: selecting the right machine learning model. step 4: training your machine learning model. You’ve taken a major step into the world of data science by understanding the core steps involved in building a machine learning model from scratch. remember, this is just the beginning.

How To Build A Machine Learning Model By Chanin Nantasenamat To build a machine learning model, follow these steps: collect and clean data: gather relevant data and clean it to remove any errors or inconsistencies. choose a model: select the most appropriate model for your data and problem. train the model: use your data to train the model to make predictions or decisions. Step 1: define your problem. before you start building a machine learning model, you need to define the problem you’re trying to solve. this involves identifying the key features of your dataset and determining what you want to predict or classify. for example, if you’re working with customer data, you might want to predict which customers. Step 7. iterate and adjust the model in production. it's often said that the formula for success when implementing technologies is to start small, think big and iterate often. even after a machine learning model is in production and you're continuously monitoring its performance, you're not done. Step 2: collect and prepare data. data is the foundation of any machine learning model. here’s how to handle it: data collection: gather relevant data from available sources. the data can come from databases, apis, web scraping, or even manual input. data cleaning: remove duplicates, handle missing values, and correct errors.

8 Steps To Build A Machine Learning Model Copyassignment Step 7. iterate and adjust the model in production. it's often said that the formula for success when implementing technologies is to start small, think big and iterate often. even after a machine learning model is in production and you're continuously monitoring its performance, you're not done. Step 2: collect and prepare data. data is the foundation of any machine learning model. here’s how to handle it: data collection: gather relevant data from available sources. the data can come from databases, apis, web scraping, or even manual input. data cleaning: remove duplicates, handle missing values, and correct errors. Machine learning models are computer programs that are used to recognize patterns in data or make predictions. machine learning models are created from machine learning algorithms, which undergo a training process using either labeled, unlabeled, or mixed data. different machine learning algorithms are suited to different goals, such as. Model building is an iterative process that follows the following basic steps: train the model: train the model on the training set using an appropriate algorithm. during training, the model.
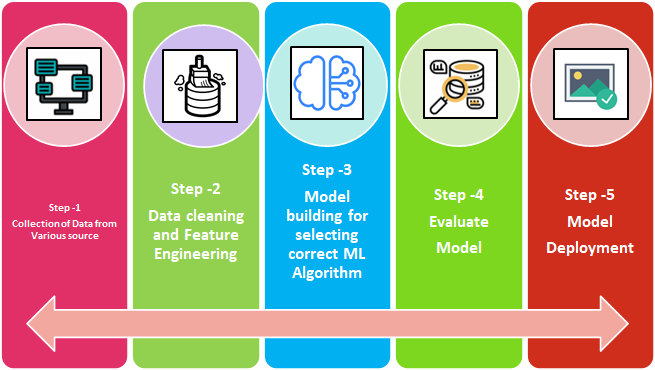
Introduction To Machine Learning Machine learning models are computer programs that are used to recognize patterns in data or make predictions. machine learning models are created from machine learning algorithms, which undergo a training process using either labeled, unlabeled, or mixed data. different machine learning algorithms are suited to different goals, such as. Model building is an iterative process that follows the following basic steps: train the model: train the model on the training set using an appropriate algorithm. during training, the model.
Comments are closed.