Kubernetes Gpus The Backbone For Ai Enabling Workloads
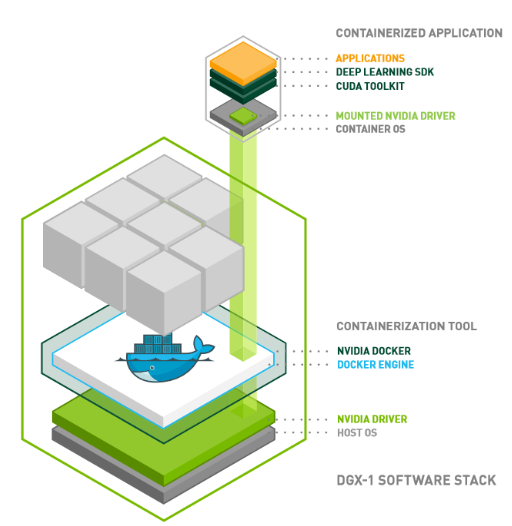
Nvidia Opens Gpus For Ai Work With Containers Kubernetes Laptrinhx That's why, in this issue, let's explore the interesting trilogy surrounding kubernetes and gpus for ai enabling workloads on large scale gpu accelerated computing together. kubernetes in 2024. This gives gpus an unfair advantage when it comes to training large ai models. kubernetes, on the other hand, is designed to scale the infrastructure by pooling the compute resources from all the nodes of the cluster, it offers unparalleled scalability for handling ai workloads, and the combination of kubernetes and gpus delivers unmatched.
Kubernetes Gpus The Backbone For Ai Enabling Workloads The rapid evolution of ai models has driven the need for more efficient and scalable inferencing solutions. as organizations strive to harness the power of ai, they face challenges in deploying, managing, and scaling ai inference workloads. nvidia nim and google kubernetes engine (gke) together offer a powerful solution to address these. Nvidia device plugin for kubernetes plays a crucial role in enabling organizations to harness the power of gpus for accelerating machine learning workloads. introduction. generative ai is having a moment right now, in no small part due to the immense scale of computing resources being leveraged to train and serve these models. About mahesh yeole mahesh yeole is an engineering manager on nvidia cloud native team, specializing in enabling gpu workloads within kubernetes environments. he is focused on enabling gpu accelerated dl and ai workloads in container orchestration systems such as kubernetes and openshift using the nvidia gpu operator. Gpus kubernetes = ? in 2024, kubernetes continues to witness widespread adoption, serving as the backbone for organizations seeking to streamline the deployment, management, and scaling of containerized applications. this surge in adoption prompts devops, platform engineering, and development teams to prioritize the reliability, security, and.
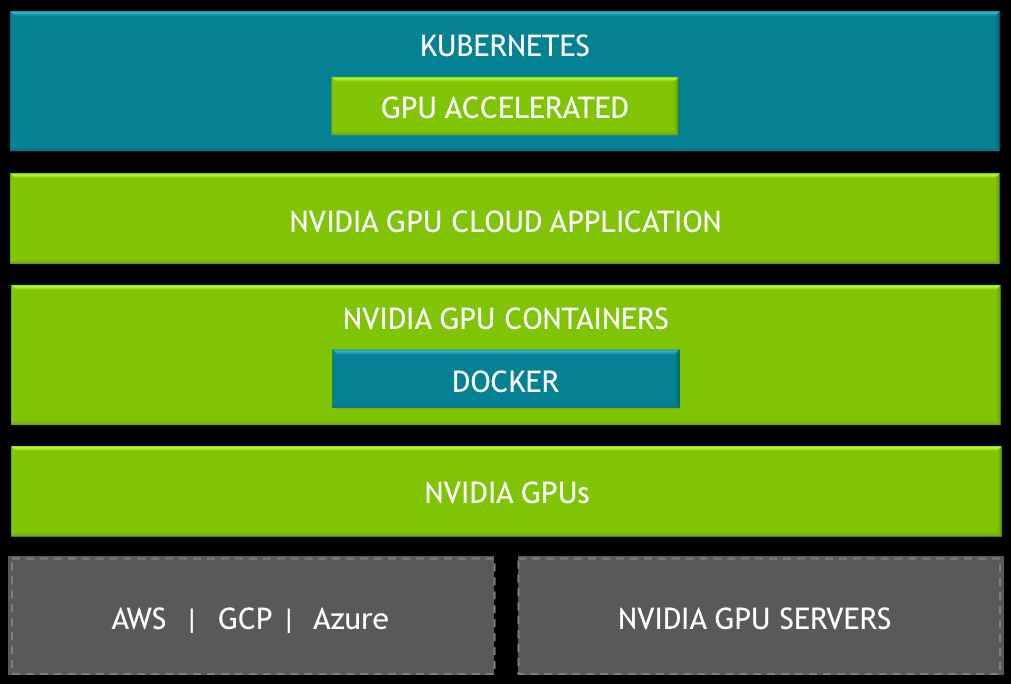
Nvidia Opens Gpus For Ai Work With Containers Kubernetes The New Stack About mahesh yeole mahesh yeole is an engineering manager on nvidia cloud native team, specializing in enabling gpu workloads within kubernetes environments. he is focused on enabling gpu accelerated dl and ai workloads in container orchestration systems such as kubernetes and openshift using the nvidia gpu operator. Gpus kubernetes = ? in 2024, kubernetes continues to witness widespread adoption, serving as the backbone for organizations seeking to streamline the deployment, management, and scaling of containerized applications. this surge in adoption prompts devops, platform engineering, and development teams to prioritize the reliability, security, and. The integration of cloud gpus within the containerized ecosystem is transforming how enterprises and ai datacenters manage, deploy, and scale applications. leveraging the power of high performance gpus, such as nvidia's h100 and h200, within a container runtime environment opens doors to faster processing, optimized workloads, and advanced machine learning capabilities in the cloud. Scalability: easily scale your gpu resources within your kubernetes environment as your ai ml workloads grow, allowing you to keep pace with your data and business needs. cost effectiveness : our competitive pricing makes ai ml development more affordable, enabling businesses of all sizes to access the tools they need to succeed in an ai driven.
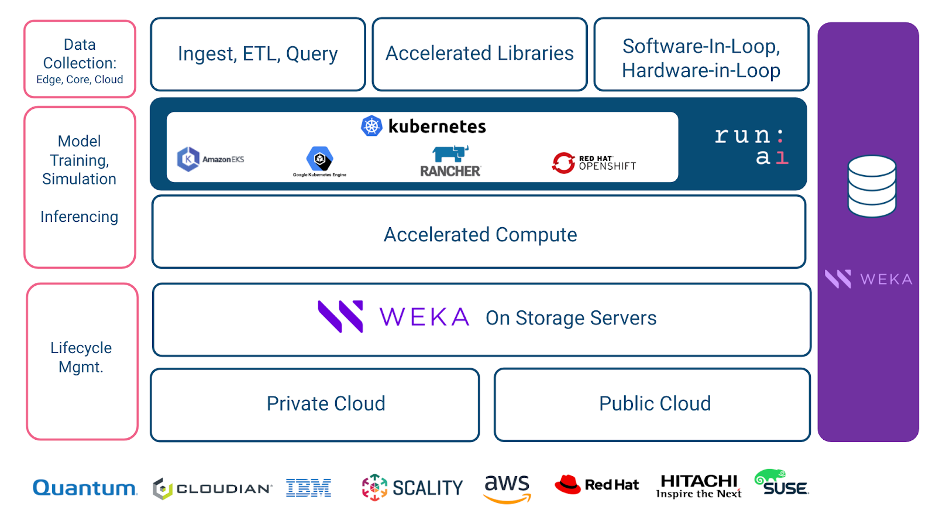
Kubernetes For Ai Ml Pipelines Using Gpus Weka The integration of cloud gpus within the containerized ecosystem is transforming how enterprises and ai datacenters manage, deploy, and scale applications. leveraging the power of high performance gpus, such as nvidia's h100 and h200, within a container runtime environment opens doors to faster processing, optimized workloads, and advanced machine learning capabilities in the cloud. Scalability: easily scale your gpu resources within your kubernetes environment as your ai ml workloads grow, allowing you to keep pace with your data and business needs. cost effectiveness : our competitive pricing makes ai ml development more affordable, enabling businesses of all sizes to access the tools they need to succeed in an ai driven.
Comments are closed.