Gpus In Kubernetes For Ai Workloads

Videogist Unlocking The Full Potential Of Gpus For Ai Workloads On About mahesh yeole mahesh yeole is an engineering manager on nvidia cloud native team, specializing in enabling gpu workloads within kubernetes environments. he is focused on enabling gpu accelerated dl and ai workloads in container orchestration systems such as kubernetes and openshift using the nvidia gpu operator. Install the nvidia gpu operator using helm. the last piece of the puzzle, we need to let kubernetes know that we have nodes with gpu’s on ’em. the nviida gpu operator creates configures manages gpus atop kubernetes and is installed with via helm chart. install helm following the official instructions.

Unlocking The Full Potential Of Gpus For Ai Workloads On Kubernetes This gives gpus an unfair advantage when it comes to training large ai models. kubernetes, on the other hand, is designed to scale the infrastructure by pooling the compute resources from all the nodes of the cluster, it offers unparalleled scalability for handling ai workloads, and the combination of kubernetes and gpus delivers unmatched. The rapid evolution of ai models has driven the need for more efficient and scalable inferencing solutions. as organizations strive to harness the power of ai, they face challenges in deploying, managing, and scaling ai inference workloads. nvidia nim and google kubernetes engine (gke) together offer a powerful solution to address these. On azure, hpc ai workload orchestration on gpus is supported on several azure services, including azure cyclecloud, azure batch and azure kuberenetes services focus of the blog post. the focus of this article will be on getting nvidia gpus managed and configured in the best way on azure kuberentes services using nvidia gpu operator. Currently, 48% of organizations use kubernetes for ai ml workloads, and the demand for such workloads also drives usage patterns on kubernetes. paperdata views the growth in the number of ai ml workloads running on kubernetes as a proof of kubernetes’ maturity. let’s look at the key technical reasons behind this trend, how ai ml workloads.
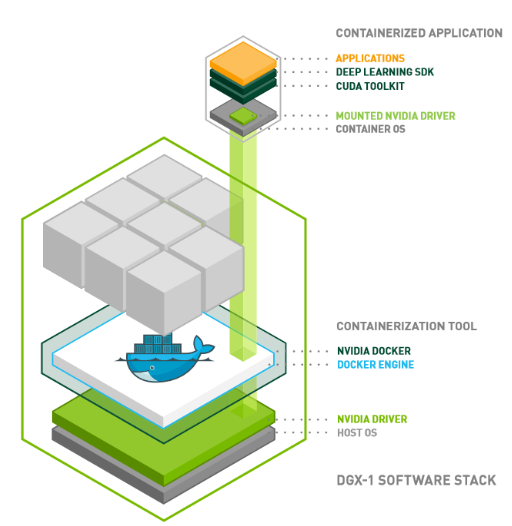
Nvidia Opens Gpus For Ai Work With Containers Kubernetes Laptrinhx On azure, hpc ai workload orchestration on gpus is supported on several azure services, including azure cyclecloud, azure batch and azure kuberenetes services focus of the blog post. the focus of this article will be on getting nvidia gpus managed and configured in the best way on azure kuberentes services using nvidia gpu operator. Currently, 48% of organizations use kubernetes for ai ml workloads, and the demand for such workloads also drives usage patterns on kubernetes. paperdata views the growth in the number of ai ml workloads running on kubernetes as a proof of kubernetes’ maturity. let’s look at the key technical reasons behind this trend, how ai ml workloads. Gpu support for ai workloads in red hat openshift 4. red hat openshift is an enterprise grade kubernetes platform for managing kubernetes clusters at scale, developed and supported by red hat. it offers a path to transform how organizations manage complex infrastructures on premises as well as across the hybrid cloud. This article describes how to efficiently run workloads that use gpu nodes on an azure kubernetes service (aks) cluster. learn how to choose the right sku, use gpu nodes to train machine learning models, and use gpu nodes to run inferences on aks. scenarios. gpu workloads can be expensive to run.
Comments are closed.