Figure 3 From Detection Of Individual Anomalous Arrival Trajectories
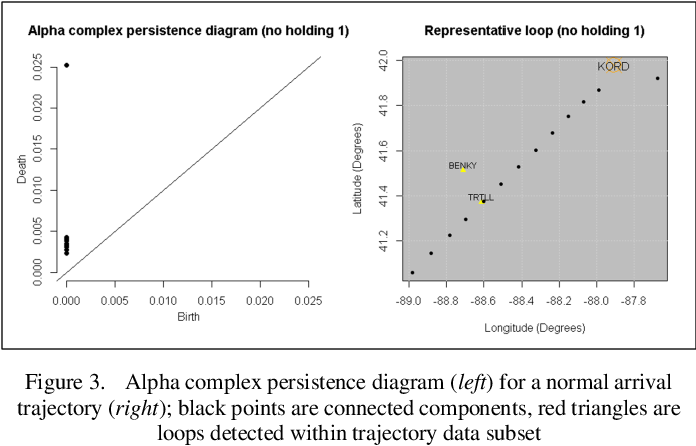
Figure 3 From Detection Of Individual Anomalous Arrival Trajectories Figure 3. alpha complex persistence diagram (left) for a normal arrival trajectory (right); black points are connected components, red triangles are loops detected within trajectory data subset "detection of individual anomalous arrival trajectories within the terminal airspace using persistent homology". However, the detection of isolated anomalous trajectories within a large aviation data set is difficult through classical methods. we propose using tools from topological data analysis to identify.
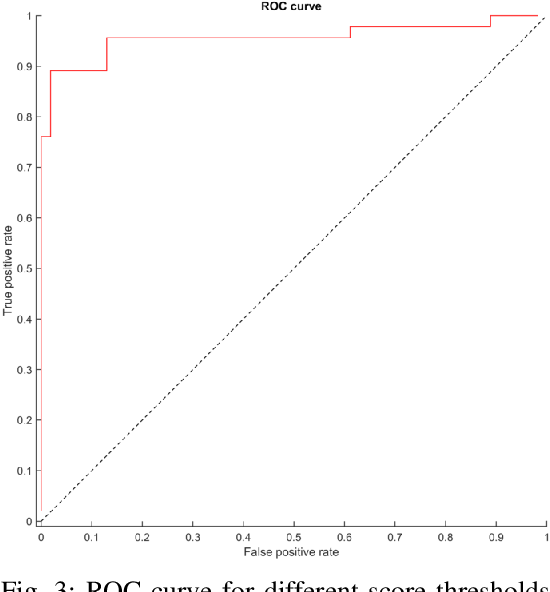
Figure 3 From Anomaly Detection In Maritime Data Based On Geometrical Figure 7. online anomalous trajectory detection results (pol data). the results show the performance of each model for the detour and random shift anomalies as we evaluate different ratios of the trajectory from 0.1 to 1.0. lm tad shows competitive results and can detect anomalies of sub trajectories. 6.5. online anomaly detection. Detailed detection count for types of anomalous: to evaluate the detection performance for each anomaly category table 3 provides the true positive detection count per category in the top 150 predictions of each dataset. this result indicates that, in most cases, our model consistently achieves high detection accuracy for anomalous with the. Identifying anomalous trajectories in the context of a user while training on all data, including anomalies. we also show that our approach is on par with state of the art methods for trajectory anomaly detection when tested on the porto dataset [32, 33], using solely gps coordinates. furthermore, our approach is suitable for online anomaly. In this paper, we propose a lightweight anomaly detection model specifically designed to detect anomalies in human trajectories. we propose a neural collaborative filtering approach to model and predict normal mobility. our method is de signed to model users’ daily patterns of life without requiring prior knowledge, thereby enhancing.
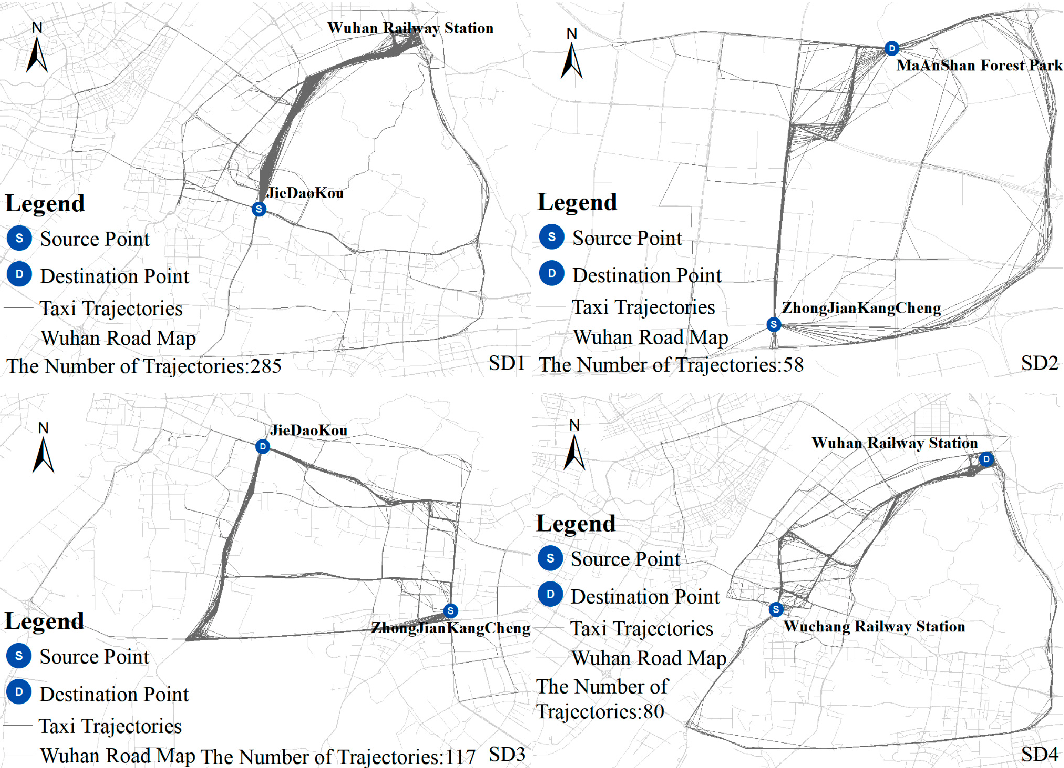
Figure 3 From Detecting Anomalous Trajectories And Behavior Patterns Identifying anomalous trajectories in the context of a user while training on all data, including anomalies. we also show that our approach is on par with state of the art methods for trajectory anomaly detection when tested on the porto dataset [32, 33], using solely gps coordinates. furthermore, our approach is suitable for online anomaly. In this paper, we propose a lightweight anomaly detection model specifically designed to detect anomalies in human trajectories. we propose a neural collaborative filtering approach to model and predict normal mobility. our method is de signed to model users’ daily patterns of life without requiring prior knowledge, thereby enhancing. Fig. 1. examples of anomalous trajectory detection. however, detecting such anomalous trajectories is non trivial due to the following two challenges: discovering normal routes. the key of trajectory anomaly detection is to effectively discover and represent the normal routes of trajectories. this is challenging because the normal. The anomaly detection task in our system is performed based on our previously proposed method iboat.in order to detect if a testing trajectory t is anomalous or not, iboat first gathers a set of trajectories t that are with the same source and destination (abbreviated as \(\langle s,d\rangle\)) pair and obtained in the similar context (similar traffic, weather condition).
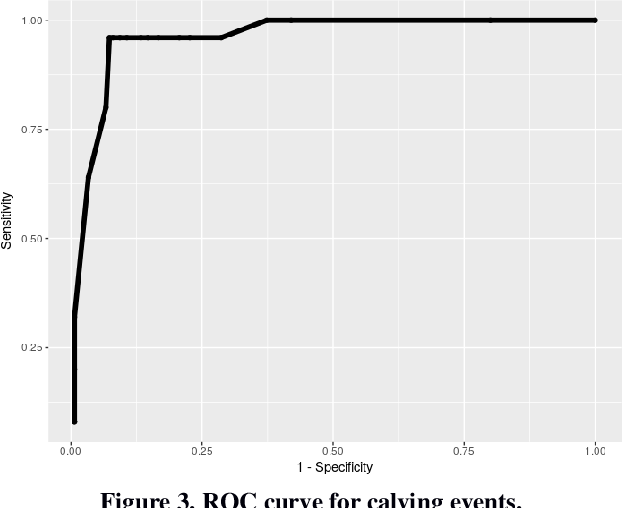
Figure 3 From Fusion Of Gps And Accelerometer Information For Anomalous Fig. 1. examples of anomalous trajectory detection. however, detecting such anomalous trajectories is non trivial due to the following two challenges: discovering normal routes. the key of trajectory anomaly detection is to effectively discover and represent the normal routes of trajectories. this is challenging because the normal. The anomaly detection task in our system is performed based on our previously proposed method iboat.in order to detect if a testing trajectory t is anomalous or not, iboat first gathers a set of trajectories t that are with the same source and destination (abbreviated as \(\langle s,d\rangle\)) pair and obtained in the similar context (similar traffic, weather condition).
Comments are closed.