Figure 1 From Anomalous Trajectory Detection And Classification Based
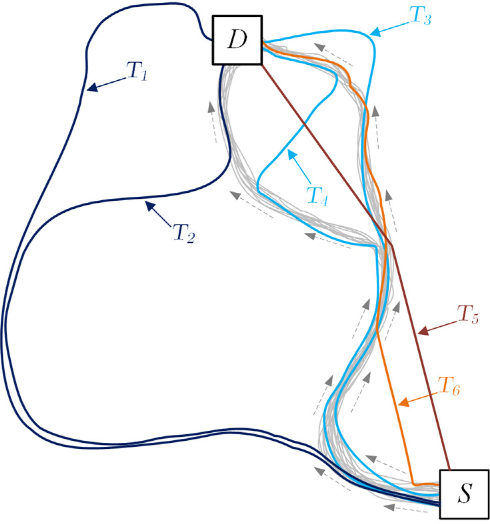
Figure 1 From Anomalous Trajectory Detection And Classification Based Anomaly detection is an important issue in trajectory data mining. various approaches have been proposed to address this issue. however, most previous studies focus only on outlier detection but rarely on pattern mining of anomalous trajectories. mining patterns of anomalous trajectories can reveal the underlying mechanisms of these outliers. this paper studies four distinct patterns of. This paper studies four distinct patterns of anomalous trajectories, and proposes a method to detect and classify them, using the difference and intersection set (dis) distance metric to evaluate the similarity between any two trajectories. anomaly detection is an important issue in trajectory data mining. various approaches have been proposed to address this issue. however, most previous.
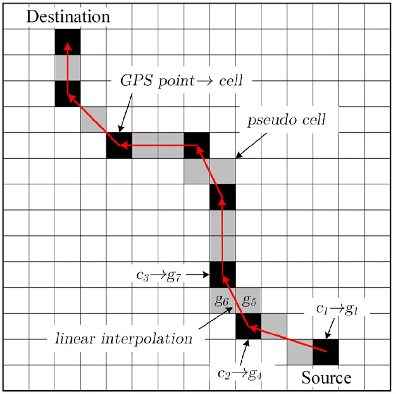
Figure 1 From Anomalous Trajectory Detection And Classification Based On of the anomaly scores used in this algorithm can be simplified. consequently, we develop a fast anomalous trajectory detec tion and classification (fastatdc) algorithm. hat reduces the time comple. ity to o(kn) where k n, by introducinga random sampling strategy. experimental results show that fastatdc achieves comparabl. Anomalous trajectory detection is a crucial task in trajectory mining fields. traditional anomalous trajectory detection methods mainly focus on the differences of a new trajectory and the historical trajectory with density and isolation techniques, which may suffer from the following two disadvantages. (1) they cannot capture the sequential information of the trajectory well. (2) they cannot. In this section, we will give a brief literature review on anomalous trajectory detection, the probability density estimation with the flow based model. 2.1. anomalous trajectory detection. trajectory anomaly detection methods can be divided into statistic based, cluster based, grid based, classification based, and deep learning methods. 2.1.1. A. trajectory anomaly detection we divide the existing studies of anomalous trajectory detection into two categories: metric based methods and learning based methods. the first category is usually based on hand crafted features and contains two steps: 1) defin ing “normal routes” with representative trajectories and 2).
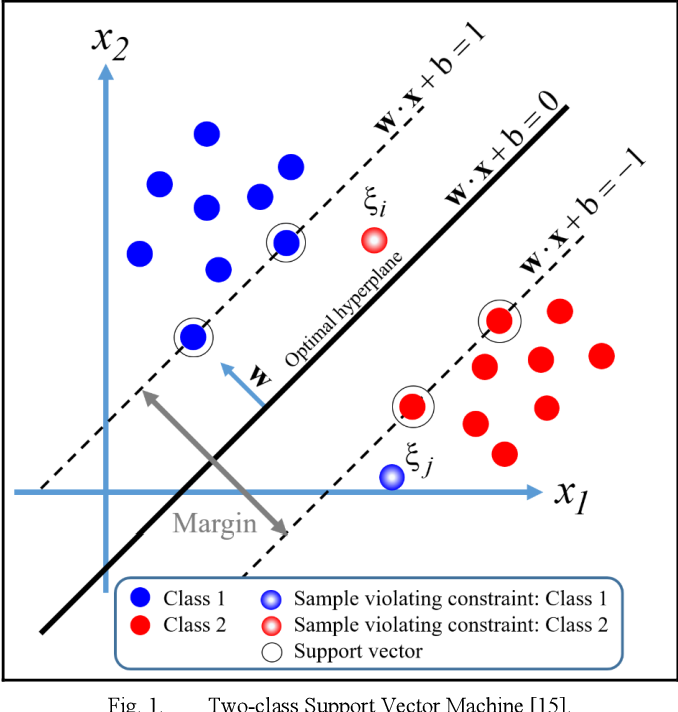
Figure 1 From Anomaly Detection Based On Gs Ocsvm Classification In this section, we will give a brief literature review on anomalous trajectory detection, the probability density estimation with the flow based model. 2.1. anomalous trajectory detection. trajectory anomaly detection methods can be divided into statistic based, cluster based, grid based, classification based, and deep learning methods. 2.1.1. A. trajectory anomaly detection we divide the existing studies of anomalous trajectory detection into two categories: metric based methods and learning based methods. the first category is usually based on hand crafted features and contains two steps: 1) defin ing “normal routes” with representative trajectories and 2). In this paper, we propose a method to detect anomalous trajectories named. anomalous trajectory detection using recurrent neural network(atd rnn). as illustrated in fig. 2, the proposed method is mainly consists of three steps, trajectory data preprocessing, trajectory embedding and anomalous detec tion. Automated detection of anomalous trajectories is an important problem with considerable applications in intelligent transportation systems. many existing studies have focused on distinguishing anomalous trajectories from normal trajectories, ignoring the large differences between anomalous trajectories. a recent study has made great progress in identifying abnormal trajectory patterns and.
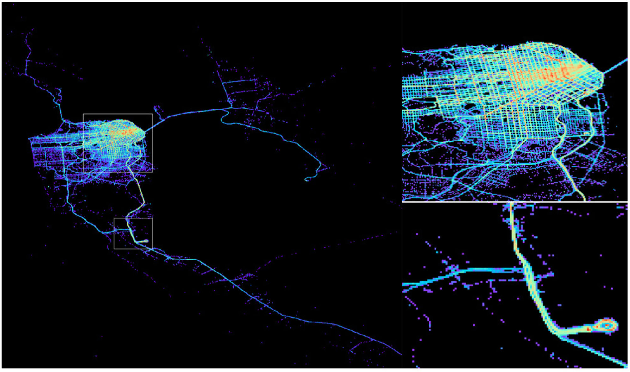
Figure 1 From Anomalous Trajectory Detection And Classification Based In this paper, we propose a method to detect anomalous trajectories named. anomalous trajectory detection using recurrent neural network(atd rnn). as illustrated in fig. 2, the proposed method is mainly consists of three steps, trajectory data preprocessing, trajectory embedding and anomalous detec tion. Automated detection of anomalous trajectories is an important problem with considerable applications in intelligent transportation systems. many existing studies have focused on distinguishing anomalous trajectories from normal trajectories, ignoring the large differences between anomalous trajectories. a recent study has made great progress in identifying abnormal trajectory patterns and.
Comments are closed.