Explainable Ai Physics In Machine Learning By Juan Nathaniel

Explainable Ai Physics In Machine Learning By Juan Nathaniel 2. physics based model that penalizes physically inconsistent output. imagine the earlier trivial case about predicting the number of goals a star footballer is going to make. if the result is below zero (ie. physically improbable), then the model could greatly penalize this prediction. Explainable ai (xai) methods can be useful in determining a neural model's relationship with data toward making it \textit{interpretable} by establishing a quantitative and tractable relationship between the input and the model's output.

Explainable Ai Physics In Machine Learning By Juan Nathaniel How artificial intelligence is revolutionizing physics research. juan nathaniel. in. towards data science. explainable ai: physics in machine learning? may 5, 2021. may 5, 2021. Neural networks are ubiquitous in high energy physics research. however, these highly nonlinear parameterized functions are treated as \\textit{black boxes} whose inner workings to convey information and build the desired input output relationship are often intractable. explainable ai (xai) methods can be useful in determining a neural model's relationship with data toward making it \\textit. Ntitative and tractable relationship between the input and the model's output. in this letter of interest, we explore the po. xai methods in the context of problems in high energy physics.1 introductionthe evolution of machine learning machine learning models in high energy physics (hep) research can be attributed to three factors (i) growing. Recent advances in artificial intelligence (ai) have led to its widespread industrial adoption, with machine learning systems demonstrating superhuman performance in a significant number of tasks.

Explainable Ai Physics In Machine Learning By Juan Nathaniel Ntitative and tractable relationship between the input and the model's output. in this letter of interest, we explore the po. xai methods in the context of problems in high energy physics.1 introductionthe evolution of machine learning machine learning models in high energy physics (hep) research can be attributed to three factors (i) growing. Recent advances in artificial intelligence (ai) have led to its widespread industrial adoption, with machine learning systems demonstrating superhuman performance in a significant number of tasks. In this work, we propose an explainable or white box ai approach [67], [68] to learn the underlying physics of high energy particle collisions. as a proof of concept, we present results of a gan trained on the final output of a gluon only parton shower, which not only reproduces the final distribution of particles but also learns the underlying showering mechanism using the complete event. Indeed, it is expected to play a crucial role én route toward explainable artificial intelligence (xai) even in the modern formalisation of the new generation of (possibly “deep”) neural networks and learning machines [2,3]. the present workshop will retain a sm perspective, mixing mathematical and theoretical physics with machine learning.
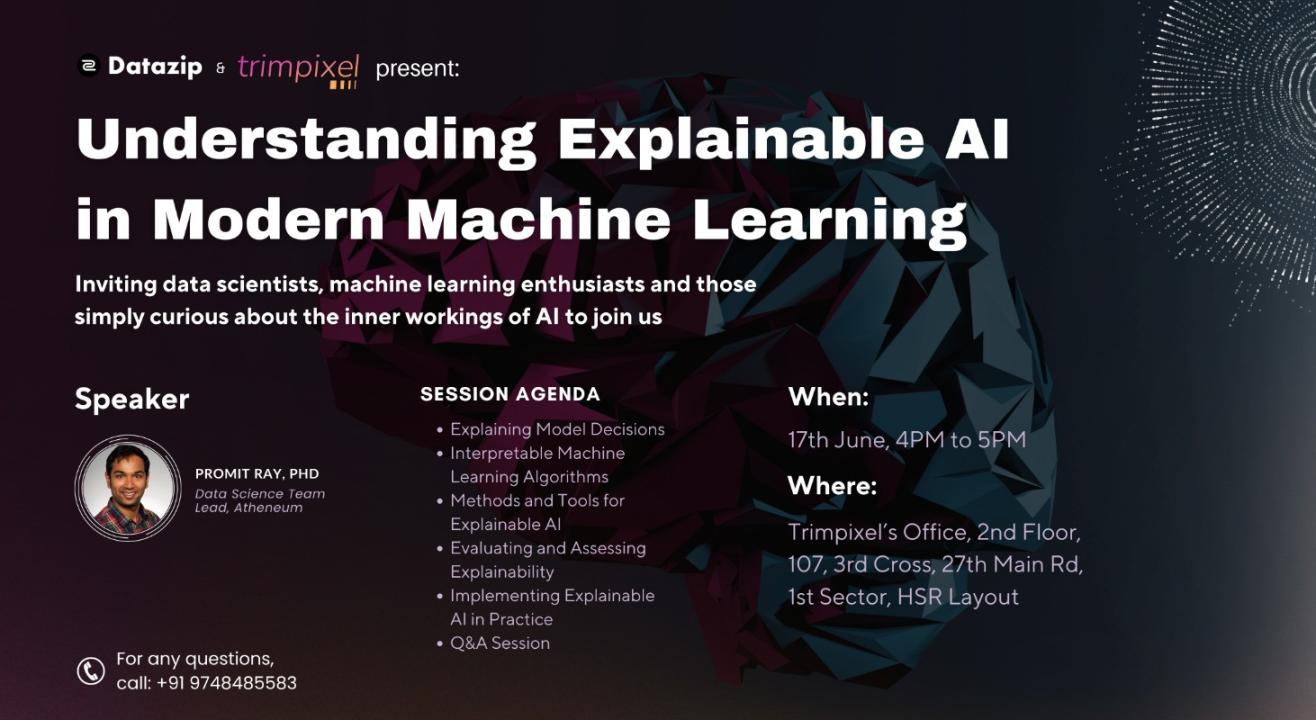
Understanding Explainable Ai In Modern Machine Learning In this work, we propose an explainable or white box ai approach [67], [68] to learn the underlying physics of high energy particle collisions. as a proof of concept, we present results of a gan trained on the final output of a gluon only parton shower, which not only reproduces the final distribution of particles but also learns the underlying showering mechanism using the complete event. Indeed, it is expected to play a crucial role én route toward explainable artificial intelligence (xai) even in the modern formalisation of the new generation of (possibly “deep”) neural networks and learning machines [2,3]. the present workshop will retain a sm perspective, mixing mathematical and theoretical physics with machine learning.
Comments are closed.