Energies Free Full Text A Review On Deep Learning Models For
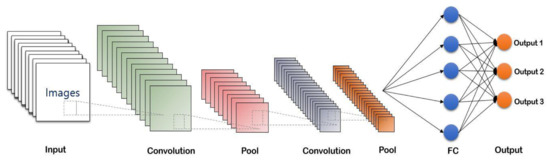
Energies Free Full Text A Review On Deep Learning Models For Presently, deep learning models are an alternative solution for predicting solar energy because of their accuracy. the present study reviews deep learning models for handling time series data to predict solar irradiance and photovoltaic (pv) power. we selected three standalone models and one hybrid model for the discussion, namely, recurrent neural network (rnn), long short term memory (lstm. Search text. search type . add circle outline et al. deep learning models for pv power forecasting: review. review" energies 17, no. 16: 3973. doi.org.
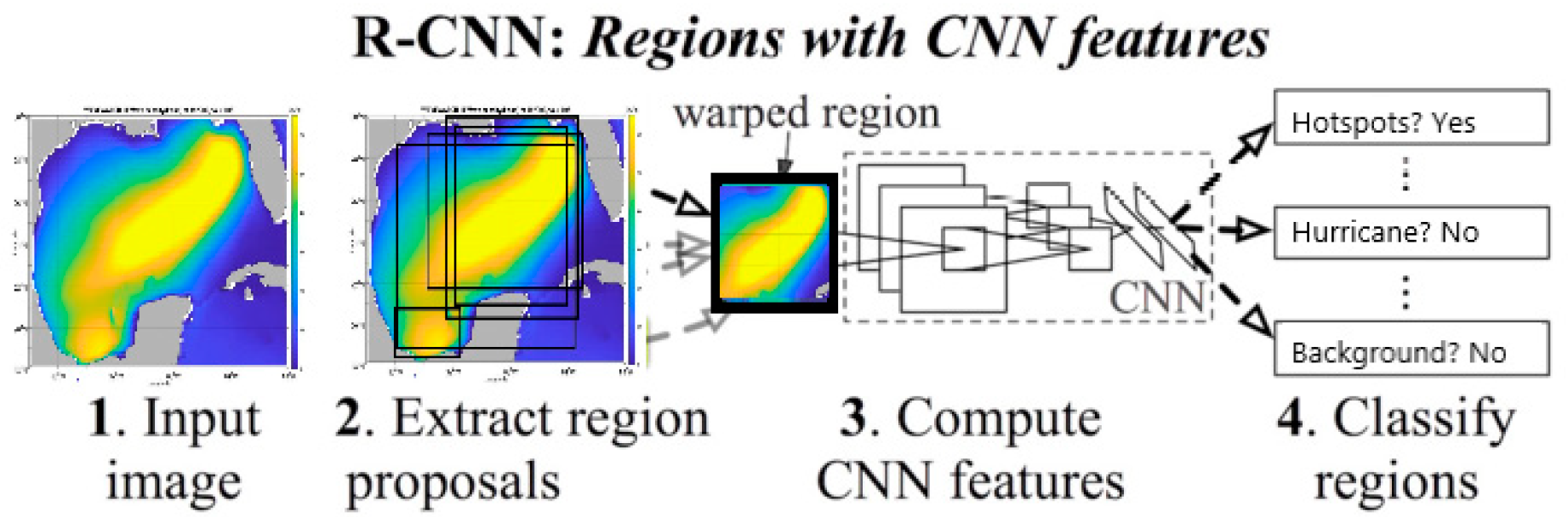
Energies Free Full Text Review On Deep Learning Research And The most significant finding is that the deep learning models of interest are more suitable for predicting solar irradiance and pv power than other conventional machine learning models. Deep learning (dl) based approaches for stlf have been referenced for a long time, considering factors such as accuracy, various performance measures, volatility, and adverse effects of uncertainties in load demand. hence, in this review, dl based studies for the stlf problem have been considered. Forecasting solar power production accurately is critical for effectively planning and managing renewable energy systems. this paper introduces and investigates novel hybrid deep learning models for solar power forecasting using time series data. the research analyzes the efficacy of various models for capturing the complex patterns present in solar power data. in this study, all of the. Download citation | on dec 1, 2022, changtian ying and others published deep learning for renewable energy forecasting: a taxonomy, and systematic literature review | find, read and cite all the.
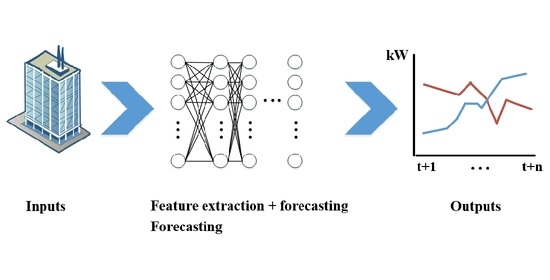
Energies Free Full Text A Review Of Deep Learning Techniques For Forecasting solar power production accurately is critical for effectively planning and managing renewable energy systems. this paper introduces and investigates novel hybrid deep learning models for solar power forecasting using time series data. the research analyzes the efficacy of various models for capturing the complex patterns present in solar power data. in this study, all of the. Download citation | on dec 1, 2022, changtian ying and others published deep learning for renewable energy forecasting: a taxonomy, and systematic literature review | find, read and cite all the. Few researchers have proposed deep hybrid models to improve the prediction performance further. a study reported that the hybrid of cnn lstm can enhance the prediction accuracy by 3.62%, 25.29%, 34.66%, 37.37% and 26.20% over cnn, lstm, gru, rnn and dnn, respectively. overall, this paper offers preliminary guidelines for a detailed view of deep. The current development in deep learning is witnessing an exponential transition into automation applications. this automation transition can provide a promising framework for higher performance and lower complexity. this ongoing transition undergoes several rapid changes, resulting in the processing of the data by several studies, while it may lead to time consuming and costly models. thus.
Comments are closed.