Electronics Free Full Text Federated Deep Reinforcement Learning

Electronics Free Full Text Node Selection Algorithm For Federated Liu l, zhao y, qi f, zhou f, xie w, he h, zheng h. federated deep reinforcement learning for joint aebss deployment and computation offloading in aerial edge computing network. electronics . 2022; 11(21):3641. In the 6g aerial network, all aerial communication nodes have computing and storage functions and can perform real time wireless signal processing and resource management. in order to make full use of the computing resources of aerial nodes, this paper studies the mobile edge computing (mec) system based on aerial base stations (aebss), proposes the joint optimization problem of computation.
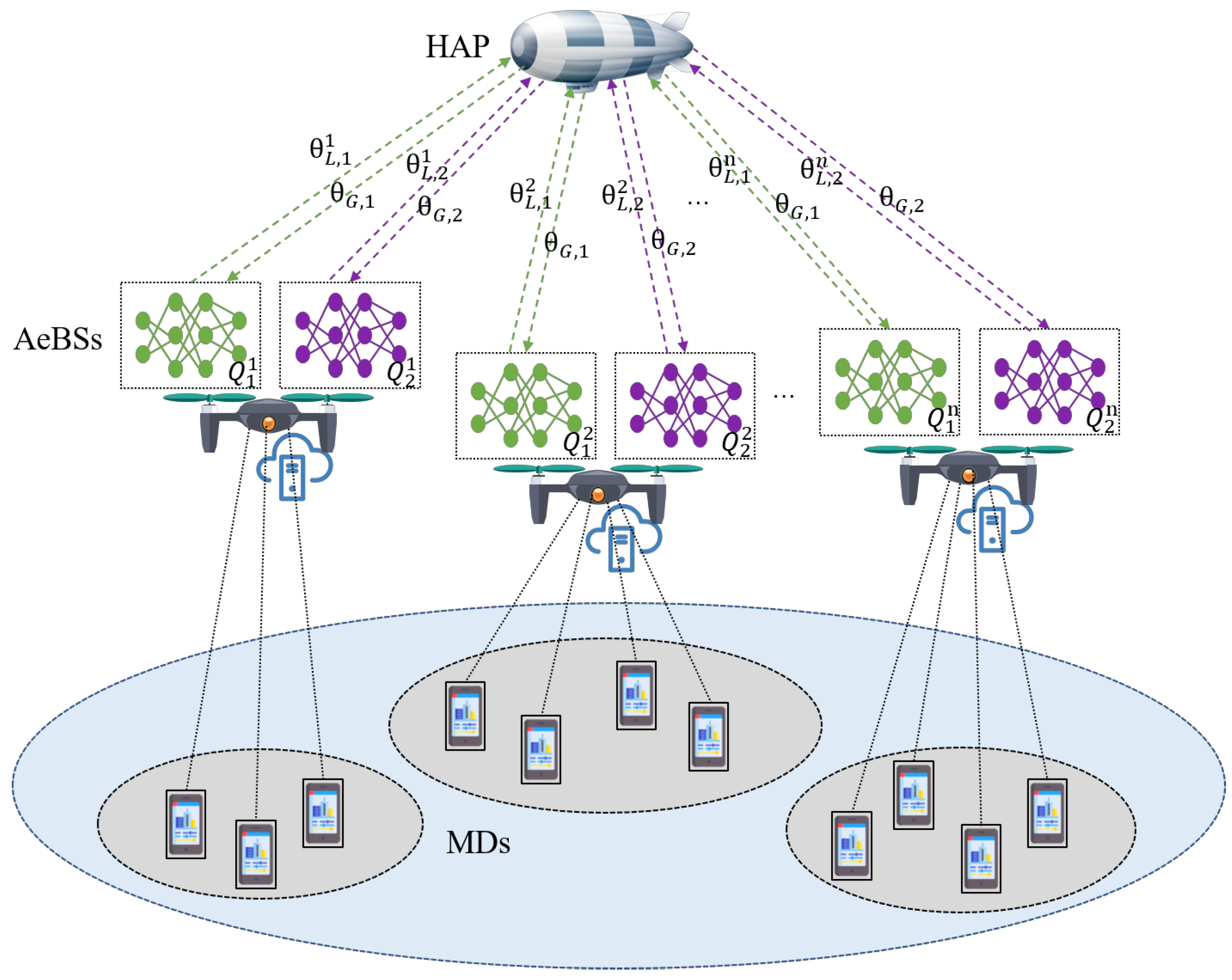
Electronics Free Full Text Federated Deep Reinforcement Learning View a pdf of the paper titled federated deep reinforcement learning, by hankz hankui zhuo and 4 other authors. in deep reinforcement learning, building policies of high quality is challenging when the feature space of states is small and the training data is limited. despite the success of previous transfer learning approaches in deep. Lee et al. [18] propose a novel federated reinforcement learning (frl) approach for the energy management of multiple smart homes with home appliances, a solar photovoltaic system, and an energy storage system. however, the current fl focus on building a global model instead of a personalized model to maximize the energy management performance. This paper presents a comprehensive survey of federated reinforcement learning (frl), an emerging and promising field in reinforcement learning (rl). starting with a tutorial of federated learning (fl) and rl, we then focus on the introduction of frl as a new method with great potential by leveraging the basic idea of fl to improve the performance of rl while preserving data privacy. according. In this paper, we investigate the problem of task scheduling in automated warehouses with hetero geneous autonomous robotic systems. we formulate the task scheduling for a heterogeneous autonomous robots (har) system in each warehouse as a queueing control optimization problem in which we aim to minimize the queue length of tasks that are waiting to be processed. we propose a deep.

Electronics Free Full Text Federated Deep Reinforcement Learning This paper presents a comprehensive survey of federated reinforcement learning (frl), an emerging and promising field in reinforcement learning (rl). starting with a tutorial of federated learning (fl) and rl, we then focus on the introduction of frl as a new method with great potential by leveraging the basic idea of fl to improve the performance of rl while preserving data privacy. according. In this paper, we investigate the problem of task scheduling in automated warehouses with hetero geneous autonomous robotic systems. we formulate the task scheduling for a heterogeneous autonomous robots (har) system in each warehouse as a queueing control optimization problem in which we aim to minimize the queue length of tasks that are waiting to be processed. we propose a deep. Evans. publishing. a review of personalized federated reinforcement learning. gaofeng chen, qingtao wu. henan university of science and technology, luoy ang 471000, china. abstract. reinforcement. 5.3 the application of deep reinforcement learning. in this work, we advocate for the use of deep reinforcement learning (drl) to handle large state action spaces and refine approximation accuracy. although dqn, a popular drl algorithm, appears in our code, it serves as a foundational concept from which our specialized drl approach is derived.

Electronics Free Full Text Federated Deep Reinforcement Learning Evans. publishing. a review of personalized federated reinforcement learning. gaofeng chen, qingtao wu. henan university of science and technology, luoy ang 471000, china. abstract. reinforcement. 5.3 the application of deep reinforcement learning. in this work, we advocate for the use of deep reinforcement learning (drl) to handle large state action spaces and refine approximation accuracy. although dqn, a popular drl algorithm, appears in our code, it serves as a foundational concept from which our specialized drl approach is derived.
Comments are closed.