Deploying Ml Models In Production An Overview

Deploying Ml Models In Production An Overview Youtube Deploying machine learning models into real world applications is a critical phase that brings theoretical models into practical use. this process involves several steps, from training and validating models to ensuring they perform well in production environments. this guide provides insights into best practices for deploying machine learning. The deployment of ml models in production is a delicate process filled with challenges. you can deploy a model via a rest api, on an edge device, or as as a.
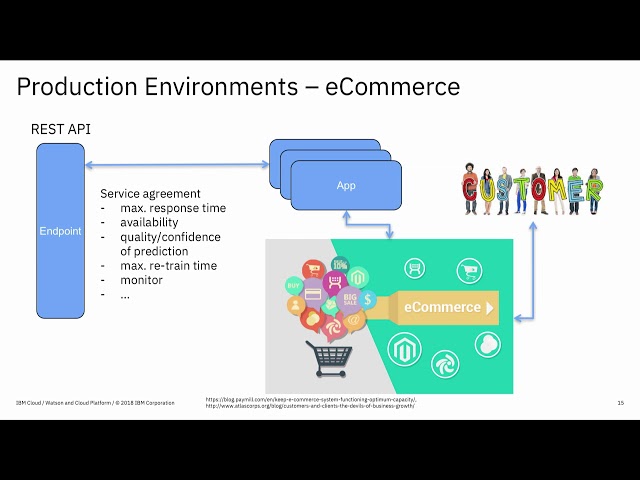
How To Deploy Machine Learning Models In Production Reason Town This article is for anyone who wants to understand how ml models are deployed in production and what strategies can be used in deploying those models. i will illustrate the general approach to deploying ml models, different strategies that can be adopted for deploying, and where these are generally implemented. Deployment involves transitioning an ml model from an offline environment into an existing production system. this step is pivotal for the model to fulfill its intended purpose and effectively tackle the challenges it was designed for. deployment establishes an online learning mechanism where the model is continuously updated with new data. How to deploy a machine learning model into production. machine learning model deployment requires an assortment of skills and talents all working together. a data science team develops the model, another team validates it and engineers are responsible for deploying it into its production environment. prepare to deploy the ml model. Ml model deployment is the critical phase where models move from theoretical constructs to practical tools that impact real world business processes. in this article, we explore the key aspects of deploying ml models, including system architecture, deployment methods, and the challenges you might face. by understanding these elements, you can.

An Introduction To Machine Learning Model Deployment How to deploy a machine learning model into production. machine learning model deployment requires an assortment of skills and talents all working together. a data science team develops the model, another team validates it and engineers are responsible for deploying it into its production environment. prepare to deploy the ml model. Ml model deployment is the critical phase where models move from theoretical constructs to practical tools that impact real world business processes. in this article, we explore the key aspects of deploying ml models, including system architecture, deployment methods, and the challenges you might face. by understanding these elements, you can. The goal of building a machine learning model is to solve a problem, and a machine learning model can only do so when it is in production and actively in use by consumers. as such, model deployment is as important as model building. as redapt points out, there can be a “disconnect between it and data science. it tends to stay focused on. Mlops stands for machine learning operations. mlops is focused on streamlining the process of deploying machine learning models to production, and then maintaining and monitoring them. mlops is a collaborative function, often consisting of data scientists, ml engineers, and devops engineers. the word mlops is a compound of two different fields.
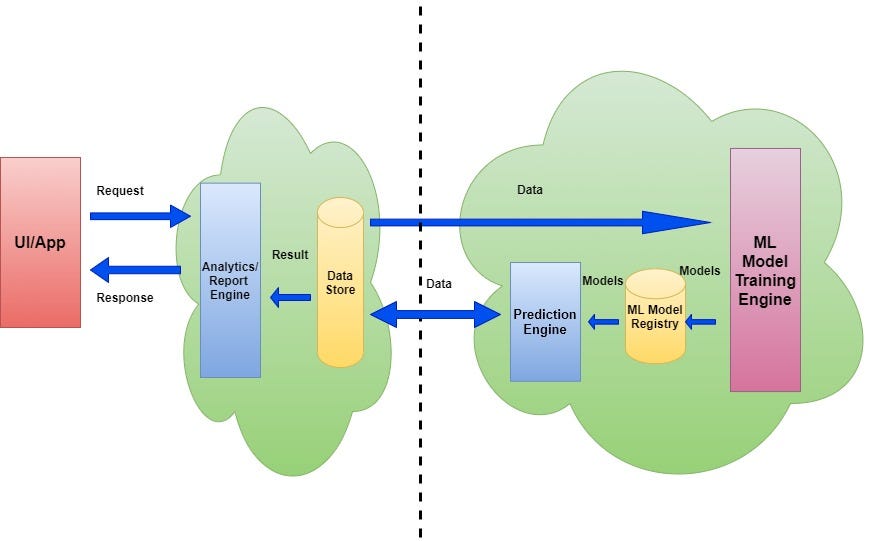
Deploying Ml Models In Production Model Export System Architecture The goal of building a machine learning model is to solve a problem, and a machine learning model can only do so when it is in production and actively in use by consumers. as such, model deployment is as important as model building. as redapt points out, there can be a “disconnect between it and data science. it tends to stay focused on. Mlops stands for machine learning operations. mlops is focused on streamlining the process of deploying machine learning models to production, and then maintaining and monitoring them. mlops is a collaborative function, often consisting of data scientists, ml engineers, and devops engineers. the word mlops is a compound of two different fields.
Comments are closed.