Deep Learning Algorithms For Morphological Classification Of Galaxies

Webinar Deep Learning Algorithms For Morphological Classification Of Classifying the morphologies of galaxies is an important step in understanding their physical properties and evolutionary histories. the advent of large scale surveys has hastened the need to develop techniques for automated morphological classification. we train and test several convolutional neural network architectures to classify the morphologies of galaxies in both a 3 class (elliptical. Although the direct cnns outperform the hierarchical classifiers, the 3 way sp first classifier offers a 5 per cent improvement in s0 classification accuracy. 4.4 galaxy morphology. galaxies exhibit an array of fine morphological structure, from spiral arms, dust lanes and stellar bars, to tidal tails and or tidal interaction (conselice 2014.

Deep Learning Algorithms For Morphological Classification Of Galaxies During the past three years, galaxy morphology classification using deep learning algorithms has received increasingly more attention. deep learning models are composed of multiple nonlinear layers to learn data representations, these layers are directly fed with raw data and automatically learn the data representations (bengio et al. 2013. View a pdf of the paper titled machine and deep learning applied to galaxy morphology a comparative study, by p. h. barchi and 9 other authors. morphological classification is a key piece of information to define samples of galaxies aiming to study the large scale structure of the universe. in essence, the challenge is to build up a robust. In observational cosmology, the morphological classification is the most basic information when creating galaxy catalogs. the first classification system, by hubble, 1926, hubble, 1936, distinguishes galaxies with dominant bulge component — also known as early type galaxies (etgs) – from galaxies with a prominent disk component – named late type galaxies (ltgs). Abstract. with the construction of large telescopes and the explosive growth of observed galaxy data, we are facing the problem to improve the data processing efficiency while ensuring the accuracy of galaxy morphology classification. therefore, this work designed a lightweight deep learning framework, efficientnet g3, for galaxy morphology.
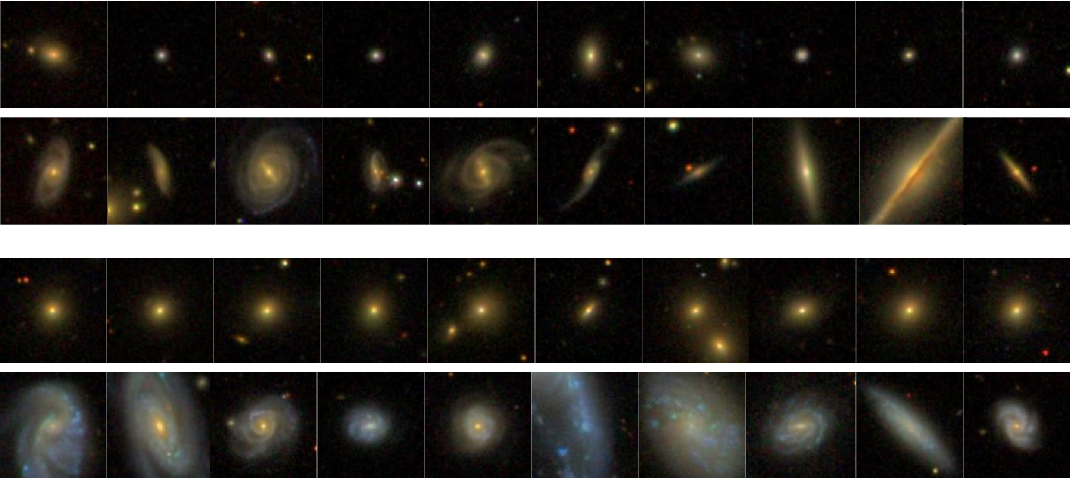
Figure 2 From Deep Learning For Morphological Classification Of In observational cosmology, the morphological classification is the most basic information when creating galaxy catalogs. the first classification system, by hubble, 1926, hubble, 1936, distinguishes galaxies with dominant bulge component — also known as early type galaxies (etgs) – from galaxies with a prominent disk component – named late type galaxies (ltgs). Abstract. with the construction of large telescopes and the explosive growth of observed galaxy data, we are facing the problem to improve the data processing efficiency while ensuring the accuracy of galaxy morphology classification. therefore, this work designed a lightweight deep learning framework, efficientnet g3, for galaxy morphology. The way forward is clearly through machine learning, although we are still learning the best ways to apply this to galaxy morphology and other areas of astronomy, e.g. star–galaxy separation (odewahn 1992; weir, fayyad & djorgovski 1995; ball et al. 2006), the galaxy zoo challenge (chou 2014), and the strong gravitational lens finding. 3 deep learning morphological classification model in this work, we apply deep learning algorithms using cnns to morphologically classify galaxy images. deep learning is a methodology which automatically learns and extracts the most relevant features (or parameters) from raw data for a given classification problem through a set of non linear.
Comments are closed.