Applying The Mlops Lifecycle Understand Mlops Needs And How They By
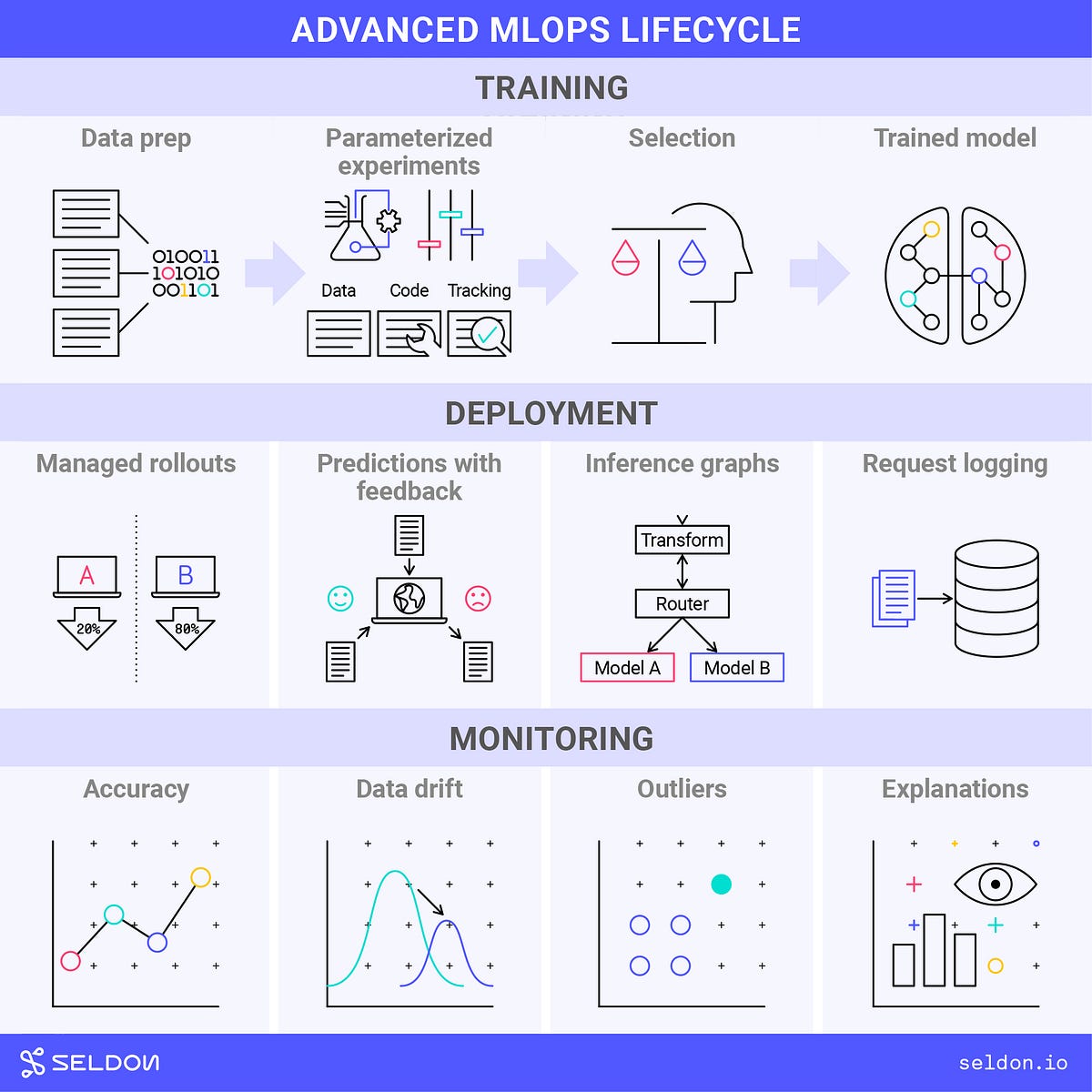
Applying The Mlops Lifecycle Understand Mlops Needs And How They By Mlops can be difficult for teams to get a grasp of. it is a new field and most teams tasked with mlops projects are currently coming at it from a different background. it is tempting to copy an approach from another project. but the needs of mlops projects can vary greatly. what is needed is to understand the specific needs of each mlops project. The mlops lifecycle refers to the structured set of stages involved in managing machine learning models in production. it encompasses data preparation, model training, evaluation, deployment, monitoring, maintenance, and retiring or replacing models. it aims to ensure the efficient and reliable operation of ml systems throughout their lifecycle.
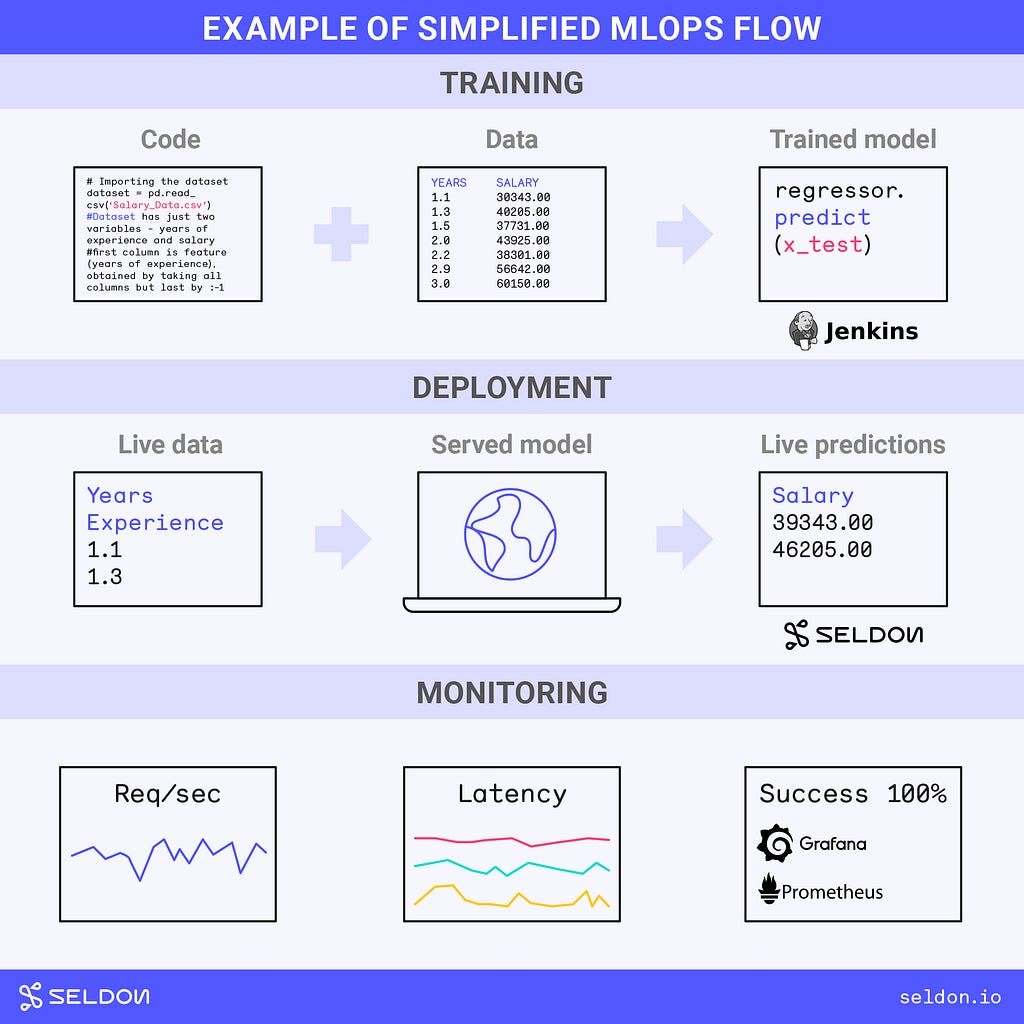
Applying The Mlops Lifecycle Laptrinhx The document is in two parts. the first part, an overview of the mlops lifecycle, is for all readers. it introduces mlops processes and capabilities and why they’re important for successful adoption of ml based systems. the second part is a deep dive on the mlops processes and capabilities. this part is for readers who want to un. Applying the mlops lifecycle. seldon. december 3, 2020. understand mlops needs and how they arise through the mlops lifecycle. apply this to better scope and tackle mlops projects. mlops can be difficult for teams to get a grasp of. it is a new field and most teams tasked with mlops projects are currently coming at it from a different background. In this article, we have explored the fundamentals of mlops, its importance, and how it can be implemented to streamline the machine learning lifecycle. by understanding and applying mlops practices, organizations can achieve faster deployment, improved model performance, and greater collaboration, ultimately driving more value from their. Mlops is best defined as "a set of tools, practices, techniques, and culture that ensure reliable and scalable deployment of machine learning systems." mlops borrows from software engineering best practices such as automation and automated testing, version control, implementation of agile principles, and data management to reduce technical debt.
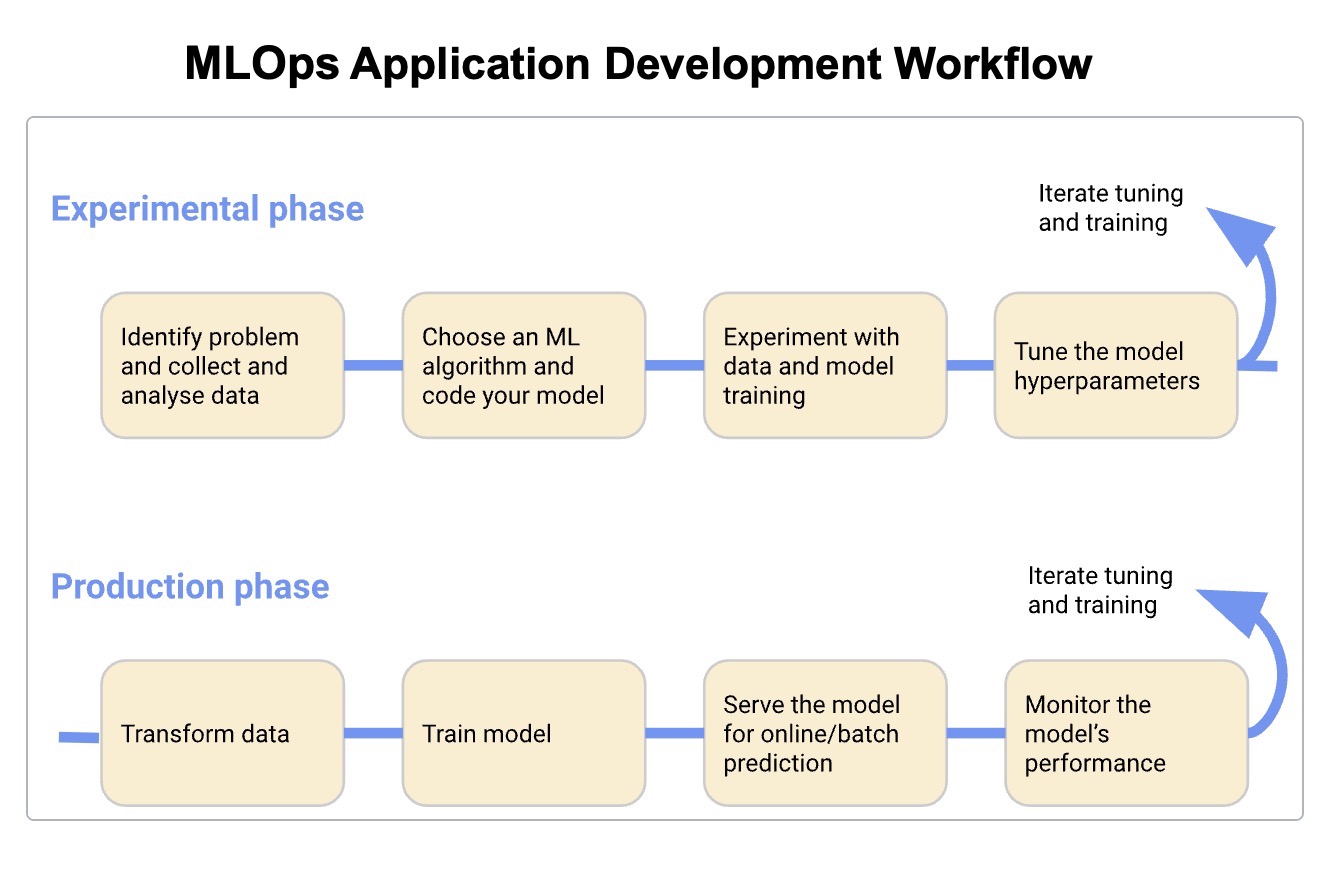
Mlops Lifecycle What Are The Stages Of Mlops Development Bitstrapped In this article, we have explored the fundamentals of mlops, its importance, and how it can be implemented to streamline the machine learning lifecycle. by understanding and applying mlops practices, organizations can achieve faster deployment, improved model performance, and greater collaboration, ultimately driving more value from their. Mlops is best defined as "a set of tools, practices, techniques, and culture that ensure reliable and scalable deployment of machine learning systems." mlops borrows from software engineering best practices such as automation and automated testing, version control, implementation of agile principles, and data management to reduce technical debt. Unifying the model and software development into one machine learning life cycle provides much needed visibility to all stakeholders. mlops lifecycle: model development and software development need to stitch together into unified machine learning life cycle. plan step is the starting point. product planning comes before everything else. This blog kicks off a series that examines the ml lifecycle, which spans (1) data and feature engineering, (2) model development, and (3) ml operations (mlops).
Mlops Lifecycle Steps Download Scientific Diagram Unifying the model and software development into one machine learning life cycle provides much needed visibility to all stakeholders. mlops lifecycle: model development and software development need to stitch together into unified machine learning life cycle. plan step is the starting point. product planning comes before everything else. This blog kicks off a series that examines the ml lifecycle, which spans (1) data and feature engineering, (2) model development, and (3) ml operations (mlops).
Comments are closed.