Applied Sciences Free Full Text A Wrapped Approach Using Unlabeled
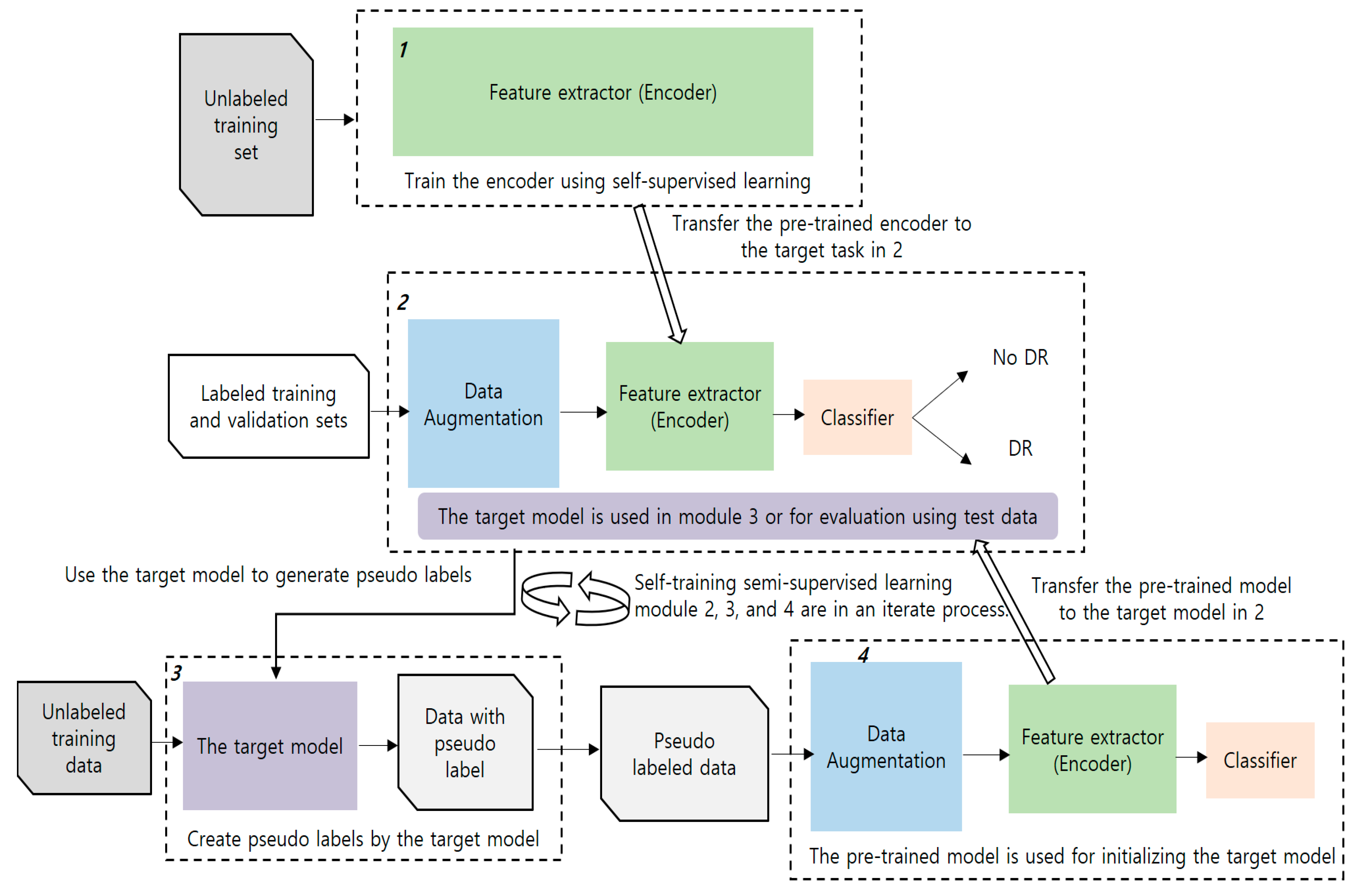
Applied Sciences Free Full Text A Wrapped Approach Using Unlabeled Biomedical text ner tagging tool with web interface for generating bert based fine tuning dataset a wrapped approach using unlabeled data for diabetic retinopathy. Large scale datasets, which have sufficient and identical quantities of data in each class, are the main factor in the success of deep learning based classification models for vision tasks. a shortage of sufficient data and interclass imbalanced data distribution, which often arise in the medical domain, cause modern deep neural networks to suffer greatly from imbalanced learning and.
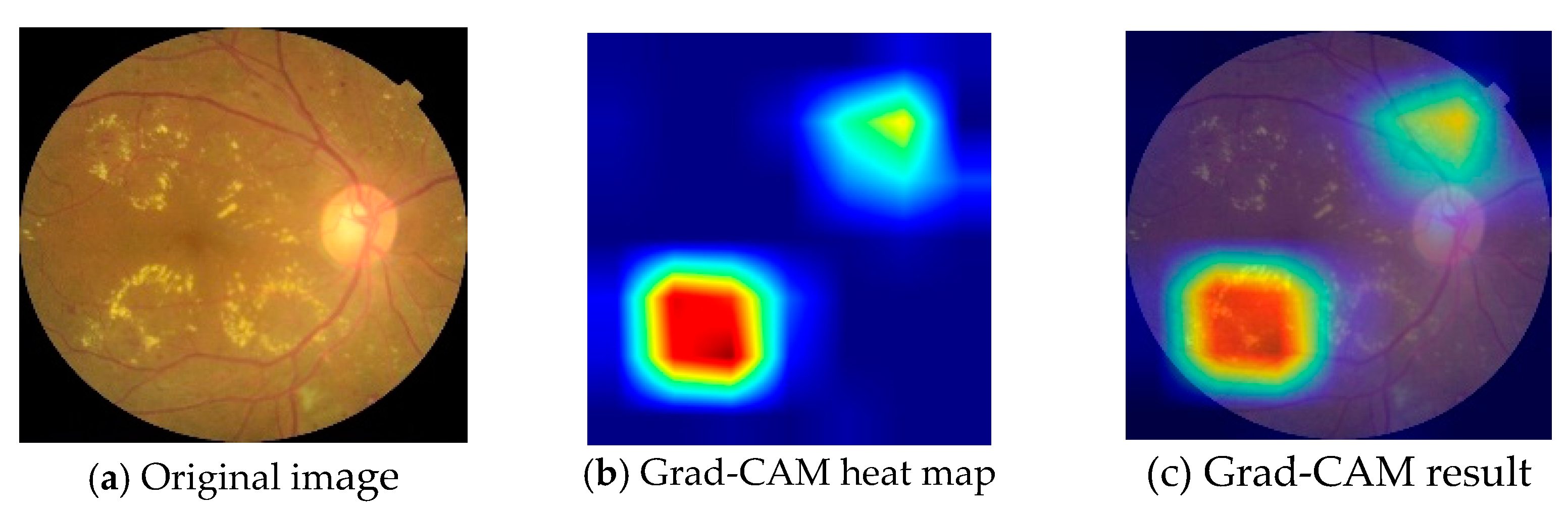
Applied Sciences Free Full Text A Wrapped Approach Using Unlabeled A wrapped approach using unlabeled data for diabetic retinopathy diagnosis. applied sciences the text of this website is published under a cc0 license. Using unlabeled data leads to a suitable improvement of accuracy in all of the models and datasets. simultaneously using knowledge distillation and unlabeled data, slightly better results are observed compared to using only unlabeled data. fig. 5, fig. 6 demonstrate that using unlabeled data could improve the training capability of a model. it. Background compared to traditional supervised machine learning approaches employing fully labeled samples, positive unlabeled (pu) learning techniques aim to classify “unlabeled” samples based on a smaller proportion of known positive examples. this more challenging modeling goal reflects many real world scenarios in which negative examples are not available—posing direct challenges to. Abstract. positive and unlabeled learning (pul) uses unlabeled documents and a few positive documents for retrieving a set of “interest” documents from a text collection. usually, pul approaches are based on the vector space model. however, when dealing with semi supervised learning for text classification or information retrieval, graph.

Applied Sciences Free Full Text A Wrapped Approach Using Unlabeled Background compared to traditional supervised machine learning approaches employing fully labeled samples, positive unlabeled (pu) learning techniques aim to classify “unlabeled” samples based on a smaller proportion of known positive examples. this more challenging modeling goal reflects many real world scenarios in which negative examples are not available—posing direct challenges to. Abstract. positive and unlabeled learning (pul) uses unlabeled documents and a few positive documents for retrieving a set of “interest” documents from a text collection. usually, pul approaches are based on the vector space model. however, when dealing with semi supervised learning for text classification or information retrieval, graph. The approach which overcomes this biased dr detection model is to add an auxiliary procedure to the target task that identifies diabetic retinopathy (dr) using supervised learning. the added process uses unlabeled data to pretrain the model that first learns features from data using self supervised or semi supervised learning, and then the. Applied sciences article a wrapped approach using unlabeled data for diabetic retinopathy diagnosis xuefeng zhang 1, youngsung kim 2, young chul chung 3, sangcheol yoon 4, sang yong rhee 5 and.
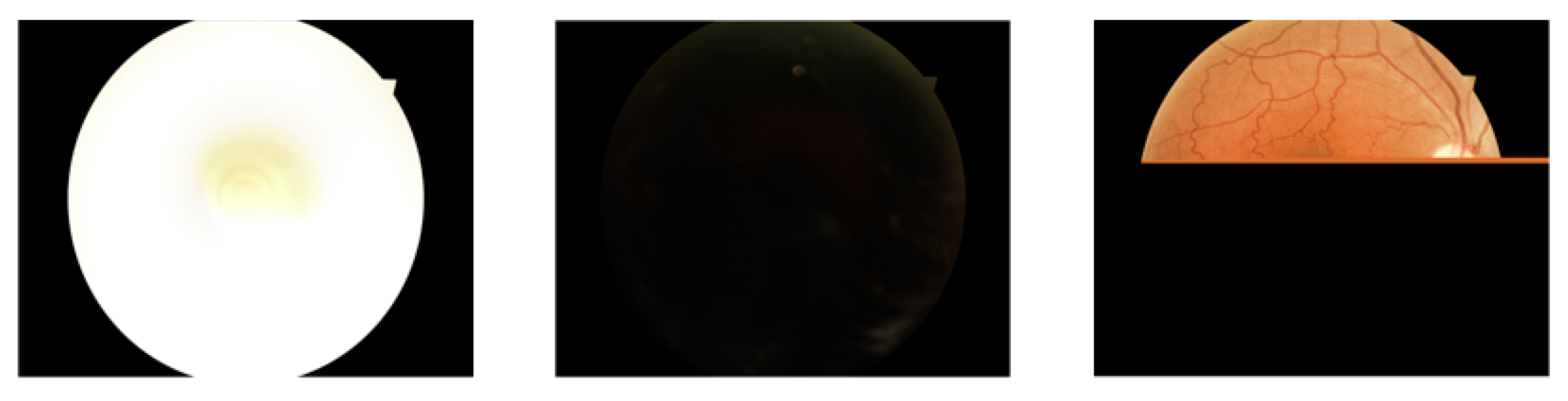
Applied Sciences Free Full Text A Wrapped Approach Using Unlabeled The approach which overcomes this biased dr detection model is to add an auxiliary procedure to the target task that identifies diabetic retinopathy (dr) using supervised learning. the added process uses unlabeled data to pretrain the model that first learns features from data using self supervised or semi supervised learning, and then the. Applied sciences article a wrapped approach using unlabeled data for diabetic retinopathy diagnosis xuefeng zhang 1, youngsung kim 2, young chul chung 3, sangcheol yoon 4, sang yong rhee 5 and.
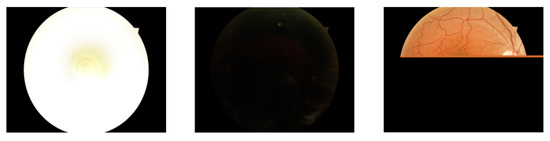
Applied Sciences Free Full Text A Wrapped Approach Using Unlabeled
Comments are closed.