A Federated Deep Reinforcement Learning Based Trust Model In Underwater Acoustic Sensor Networks
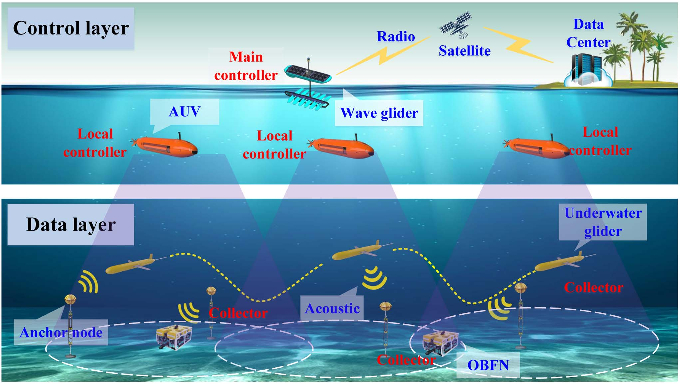
Figure 1 From A Federated Deep Reinforcement Learning Based Trust Model Underwater acoustic sensor networks (uasns) have been widely deployed in many areas, such as marine ranching, naval applications, and marine disaster warning systems. the security of uasns, particularly insider threats, is of growing concern. internal attacks carried out via compromised normal nodes are more damaging and stealthy than external attacks, such as signal stealing, data decryption. However, traditional trust models lack sufficient scalability when faced with movable underwater devices, heterogeneous network environments, and variable attack patterns. therefore, in this paper, a novel trust model based on federated deep reinforcement learning is proposed for uasns.
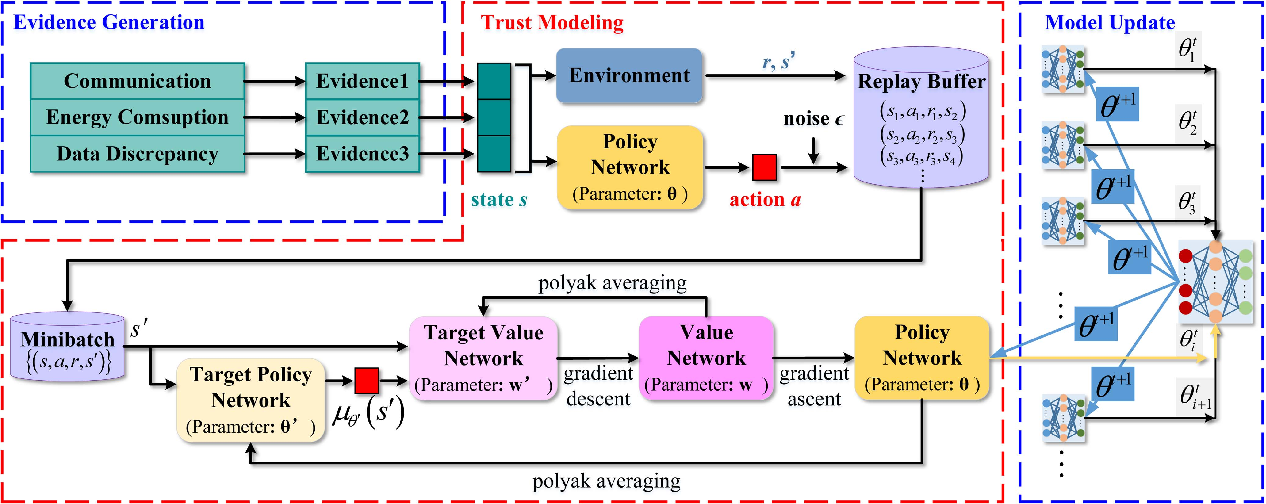
Figure 2 From A Federated Deep Reinforcement Learning Based Trust Model Training. finally, a federated learning based update method periodically aggregates and updates the parameters of the local models. the experimental results prove that the proposed scheme exhibits satisfactory performance in terms of improving trust prediction accuracy and energy efficiency. index terms—underwater acoustic sensor networks. A novel trust model based on federated deep reinforcement learning is proposed for uasns, and experimental results prove that the proposed scheme exhibits satisfactory performance in terms of improving trust prediction accuracy and energy efficiency. underwater acoustic sensor networks (uasns) have been widely deployed in many areas, such as marine ranching, naval applications, and marine. Therefore, in this paper, a novel trust model based on federated deep reinforcement learning is proposed for uasns. first, the evidence acquisition mechanism, including communication, energy, and. Second, acquired trust evidence is fed into the corresponding deep reinforcement learning based local trust model to accomplish trust prediction and model training. finally, a federated learning based update method periodically aggregates and updates the parameters of the local models. the experimental results prove that the proposed scheme.
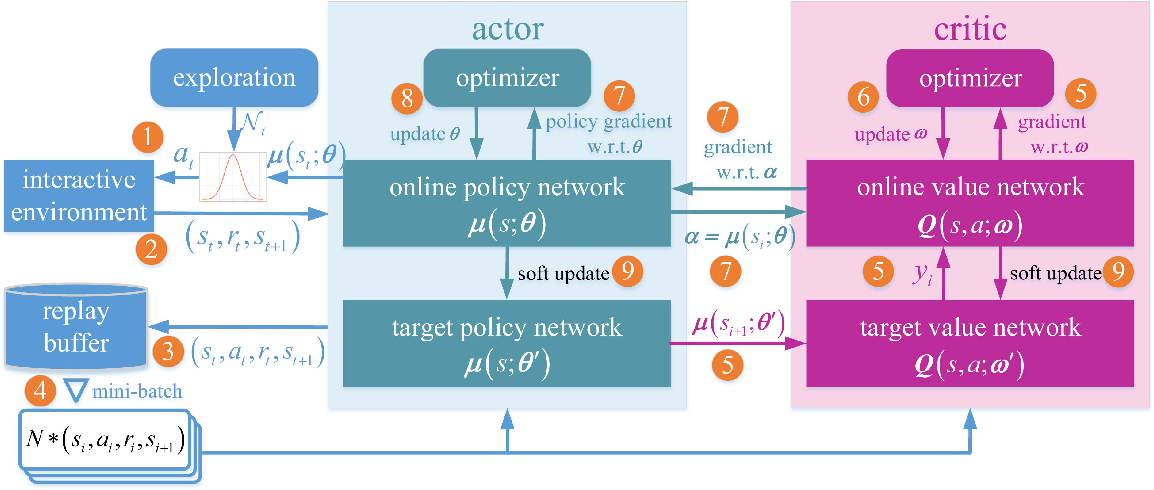
Figure 5 From A Federated Deep Reinforcement Learning Based Trust Model Therefore, in this paper, a novel trust model based on federated deep reinforcement learning is proposed for uasns. first, the evidence acquisition mechanism, including communication, energy, and. Second, acquired trust evidence is fed into the corresponding deep reinforcement learning based local trust model to accomplish trust prediction and model training. finally, a federated learning based update method periodically aggregates and updates the parameters of the local models. the experimental results prove that the proposed scheme. A federated deep reinforcement learning based trust model in underwater acoustic sensor networks. underwater acoustic sensor networks (uasns) have been widely deployed in many areas, such as marine ranching, naval applications, and marine disaster warning systems. the security of uasns, particularly insider threats, is of growing concern. Underwater acoustic sensor networks (uasns) have been widely applied in marine scenarios, such as offshore exploration, auxiliary navigation and marine military. due to the limitations in communication, computation, and storage of underwater sensor nodes, traditional security mechanisms are not applicable to uasns. recently, various trust models have been investigated as effective tools.
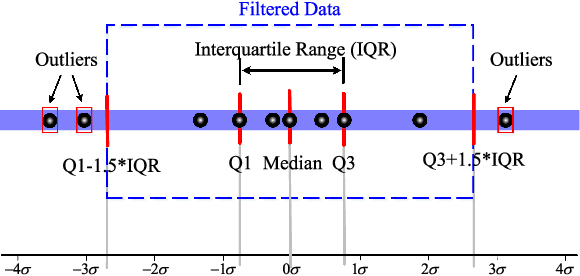
Figure 4 From A Federated Deep Reinforcement Learning Based Trust Model A federated deep reinforcement learning based trust model in underwater acoustic sensor networks. underwater acoustic sensor networks (uasns) have been widely deployed in many areas, such as marine ranching, naval applications, and marine disaster warning systems. the security of uasns, particularly insider threats, is of growing concern. Underwater acoustic sensor networks (uasns) have been widely applied in marine scenarios, such as offshore exploration, auxiliary navigation and marine military. due to the limitations in communication, computation, and storage of underwater sensor nodes, traditional security mechanisms are not applicable to uasns. recently, various trust models have been investigated as effective tools.
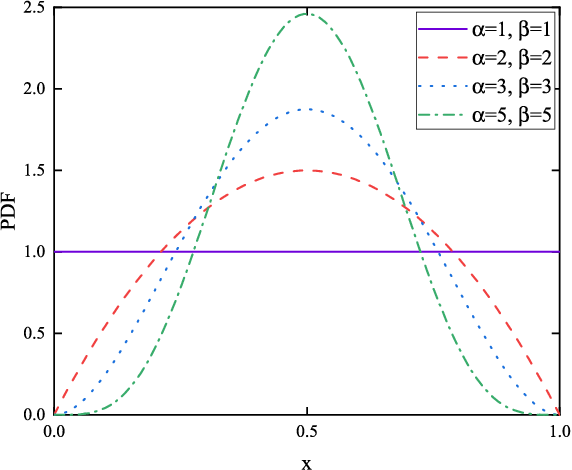
Figure 3 From A Federated Deep Reinforcement Learning Based Trust Model
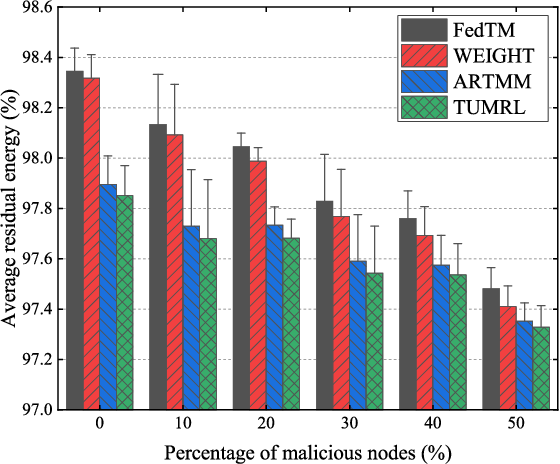
Figure 12 From A Federated Deep Reinforcement Learning Based Trust
Comments are closed.